A traffic incident detection method based on depth learning and entropy model
A traffic incident and deep learning technology, applied in the field of intelligent transportation, can solve the problems of the influence of detection links, many false detections and missed detections, and low accuracy, and achieve the effect of fast detection speed, reduction of false detection rate, and low false detection rate.
- Summary
- Abstract
- Description
- Claims
- Application Information
AI Technical Summary
Problems solved by technology
Method used
Image
Examples
Embodiment 1
[0099] For large-scale traffic jams, the spatial global feature extracts the velocity field, so the distribution of the velocity field is relatively concentrated. The spatial entropy value is small and the continuous multi-frame entropy value is stable, so the mean , variance Var are small. The probability of a traffic jam event is inversely proportional to the size and stability of the entropy value, so the mean value , The inverse coefficient of the variance Var. In terms of time, within a period of time, most video frames or sub-segments are identified as congestion event types by the CNN network; in terms of time, the distribution of event types is concentrated on the congestion category, the entropy value in the time domain is small, and the event probability larger. Construct credibility parameters:
[0100]
Embodiment 2
[0102] For a local crash event, select the continuous multi-frame speed of a feature point on a specific object in a video sub-segment as the local feature in the time domain, then the speed of the continuous multi-frame will appear scattered in size and direction. The local time entropy value is large and unstable, so the mean , variance Var are larger. The probability of a local crash event is proportional to the size and stability of the entropy value, so the mean value , Proportional coefficient of variance Var. In terms of time, most of the video frames or sub-segments are recognized by the CNN network as crash event types, and the distribution of event types in time is concentrated in the crash event category. The entropy value in the time domain is small, and the event possibility larger. Construct credibility parameters:
[0103]
[0104] Combining the joint judgment of the entropy value in space and time, and the classification results of deep learning to co...
PUM
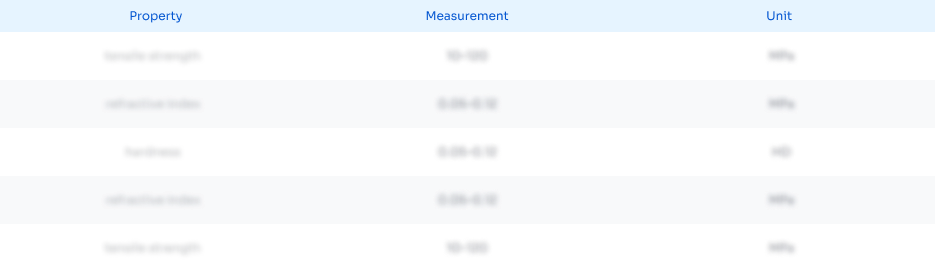
Abstract
Description
Claims
Application Information
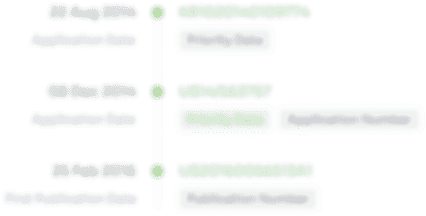
- Generate Ideas
- Intellectual Property
- Life Sciences
- Materials
- Tech Scout
- Unparalleled Data Quality
- Higher Quality Content
- 60% Fewer Hallucinations
Browse by: Latest US Patents, China's latest patents, Technical Efficacy Thesaurus, Application Domain, Technology Topic, Popular Technical Reports.
© 2025 PatSnap. All rights reserved.Legal|Privacy policy|Modern Slavery Act Transparency Statement|Sitemap|About US| Contact US: help@patsnap.com