Nonlinear system state estimation method based on Kalman filtering positioning
A Kalman filter and nonlinear system technology, applied in the field of nonlinear system state estimation based on Kalman filter positioning, can solve problems such as large root mean square error and inaccurate state estimation
- Summary
- Abstract
- Description
- Claims
- Application Information
AI Technical Summary
Problems solved by technology
Method used
Image
Examples
Embodiment 1
[0060] refer to figure 2 , the implementation steps of the strongly adaptive Kalman filter design invention for the SCKF algorithm are as follows:
[0061] Step 1. Initialize filtering
[0062] Define the initial value of the state vector of the system at k=0 Its covariance matrix is P 0|0 , get the square root S of the variance matrix by QR decomposition 0|0 , simulating and generating the RSSI values of the beacon nodes within n communication ranges received by the node to be positioned;
[0063] Step 2. Loop through SCKF
[0064] For k=1,2,...N, N is the number of samples, the obtained in step 2.2 and the S obtained in step 2.1 k|k As an input, repeat steps 2.1 and 2.2 until N samples are completed.
[0065] According to formula (5), considering that the state vector of the node to be located at time k satisfies Estimated by this order, the estimated value obtained by SCKF at this time and The error between should conform to an additive noise relationshi...
PUM
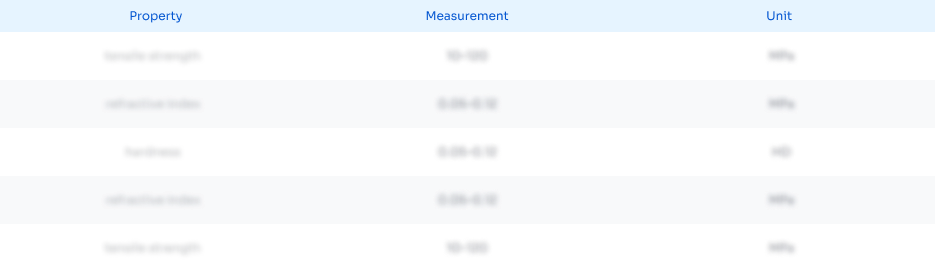
Abstract
Description
Claims
Application Information
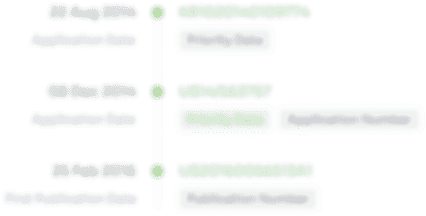
- R&D Engineer
- R&D Manager
- IP Professional
- Industry Leading Data Capabilities
- Powerful AI technology
- Patent DNA Extraction
Browse by: Latest US Patents, China's latest patents, Technical Efficacy Thesaurus, Application Domain, Technology Topic, Popular Technical Reports.
© 2024 PatSnap. All rights reserved.Legal|Privacy policy|Modern Slavery Act Transparency Statement|Sitemap|About US| Contact US: help@patsnap.com