Multimodal brain network feature fusion method based on multi-task learning
A multi-task learning and feature fusion technology, which is applied in the field of multi-modal brain network feature fusion based on multi-task learning, can solve problems such as single mode, no consideration of the relationship between modes, little functional information and structural information fusion, etc. , to improve the accuracy
- Summary
- Abstract
- Description
- Claims
- Application Information
AI Technical Summary
Problems solved by technology
Method used
Image
Examples
Embodiment Construction
[0014] The present invention will be further described below in conjunction with the accompanying drawings and specific examples.
[0015] This implementation takes the data of patients with severe depression as an example. The specific data were collected in the Second Xiangya Hospital of Central South University. Depression was diagnosed with the DSM-IV scale. For example, images were scanned with a 1.5TGE MRI scanner, and resting-state functional MRI images and diffusion tensor imaging data were acquired.
[0016] The specific implementation process of the multi-modal brain network feature fusion method based on multi-task learning of the present invention is as follows: figure 1 shown, including the following steps:
[0017] A. Preprocessing the acquired fMRI images, the specific steps are as follows:
[0018] 1. Perform data conversion and convert the original Dicom image into a NIfTI image;
[0019] Here, NIfTI is a typical data analysis format that includes some impo...
PUM
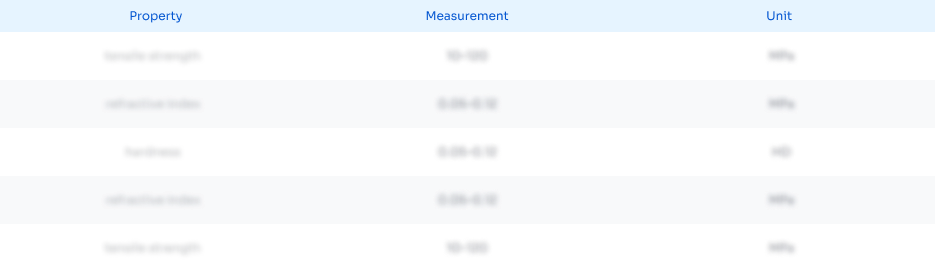
Abstract
Description
Claims
Application Information
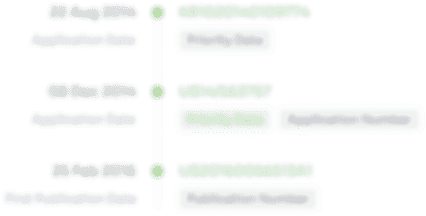
- R&D Engineer
- R&D Manager
- IP Professional
- Industry Leading Data Capabilities
- Powerful AI technology
- Patent DNA Extraction
Browse by: Latest US Patents, China's latest patents, Technical Efficacy Thesaurus, Application Domain, Technology Topic, Popular Technical Reports.
© 2024 PatSnap. All rights reserved.Legal|Privacy policy|Modern Slavery Act Transparency Statement|Sitemap|About US| Contact US: help@patsnap.com