Depth estimation method combined with semantic edge
A depth estimation and semantic technology, applied in the field of computer vision, can solve the problems of loss reliability, lack of theoretical support, complex network, etc., to achieve the effect of improving accuracy and realizing implicit loss monitoring
- Summary
- Abstract
- Description
- Claims
- Application Information
AI Technical Summary
Problems solved by technology
Method used
Image
Examples
Embodiment Construction
[0023] The present invention will be further described below in conjunction with specific embodiments. It should be understood that these examples are only used to illustrate the present invention and not to limit the scope of the present invention. In addition, it should be understood that after reading the teaching content of the present invention, those skilled in the art can make various changes or modifications to the present invention, and these equivalent forms also fall within the scope defined by the appended claims of the present application.
[0024] Embodiments of the present invention relate to a method for depth estimation combined with semantic edges, comprising the following steps: acquiring an image to be depth-estimated; inputting the image into a trained deep learning network to obtain a depth prediction map and a semantic edge prediction map;
[0025] like figure 1 As shown, the deep learning network includes: a shared feature extraction module, a depth es...
PUM
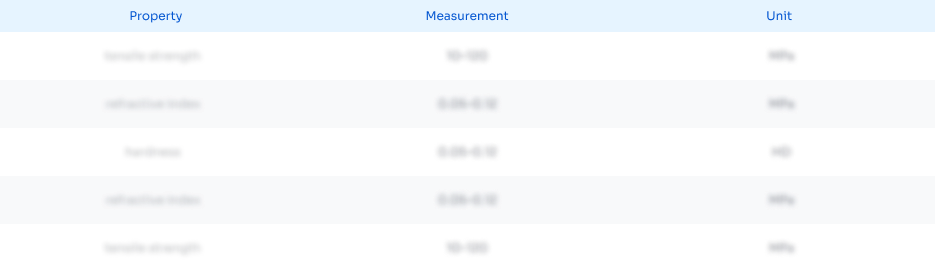
Abstract
Description
Claims
Application Information
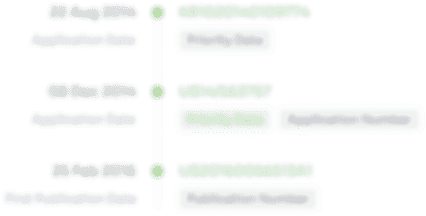
- R&D Engineer
- R&D Manager
- IP Professional
- Industry Leading Data Capabilities
- Powerful AI technology
- Patent DNA Extraction
Browse by: Latest US Patents, China's latest patents, Technical Efficacy Thesaurus, Application Domain, Technology Topic, Popular Technical Reports.
© 2024 PatSnap. All rights reserved.Legal|Privacy policy|Modern Slavery Act Transparency Statement|Sitemap|About US| Contact US: help@patsnap.com