Method for monitoring tool wear state through enhanced comparative learning of Gramer angle field
A Graham angle field and tool wear technology, which is applied in manufacturing tools, measuring/indicating equipment, metal processing equipment, etc., can solve problems such as difficult selection of basis function or kernel function parameters, limited label samples, and neural network overfitting , to achieve the effect of overcoming good performance and reducing experiment cost
- Summary
- Abstract
- Description
- Claims
- Application Information
AI Technical Summary
Problems solved by technology
Method used
Image
Examples
Embodiment 1
[0045] Example 1: A Gramm angle field enhanced contrast learning monitoring method for tool wear status, such as figure 1 shown, including the following steps:
[0046]Step 1. Collect cutting force signals in three directions and their corresponding tool wear states during the cutting process of the tool, and obtain labeled samples and non-labeled samples. The labeled samples are composed of the cutting force signals and The tool wear state is composed of; the unlabeled sample is composed of the cutting force signal in the intermediate stage of tool processing; the device collected in this embodiment is a TCM experimental device, and the tool wear state is that the tool is milled ten times on the workpiece surface, The length of each milling is 1.5m, each milling includes three forward milling and two reverse milling, and the sampling frequency is 12000Hz. After each milling is completed, the tool is removed and the tool microscope is used to take a wear picture; the tool flan...
PUM
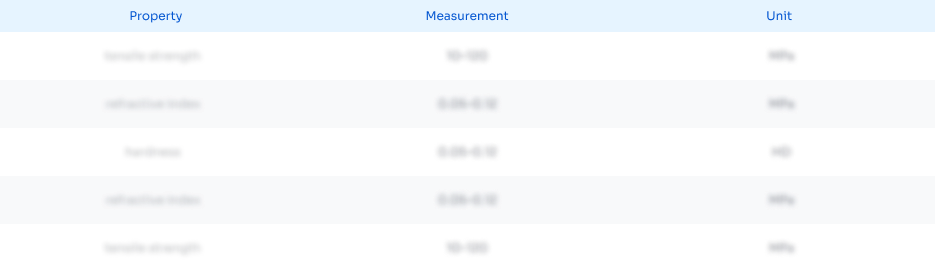
Abstract
Description
Claims
Application Information
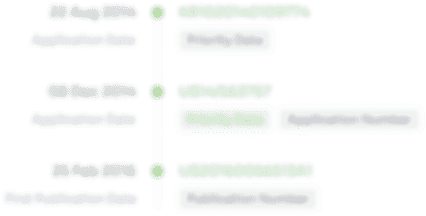
- R&D Engineer
- R&D Manager
- IP Professional
- Industry Leading Data Capabilities
- Powerful AI technology
- Patent DNA Extraction
Browse by: Latest US Patents, China's latest patents, Technical Efficacy Thesaurus, Application Domain, Technology Topic, Popular Technical Reports.
© 2024 PatSnap. All rights reserved.Legal|Privacy policy|Modern Slavery Act Transparency Statement|Sitemap|About US| Contact US: help@patsnap.com