Shield tunneling parameter prediction method based on geological parameter quantification
A technology of excavation parameters and geological parameters, applied in neural learning methods, neural architecture, design optimization/simulation, etc., can solve problems such as small application scope, differences, and inability to reflect geological conditions, and achieve the effect of improving prediction accuracy
- Summary
- Abstract
- Description
- Claims
- Application Information
AI Technical Summary
Problems solved by technology
Method used
Image
Examples
Embodiment
[0037] as Figure 1 As shown, the present embodiment provides a shield boring parameter prediction method based on geological parameter quantification, the method comprising the following steps: the formation along the direction of burial depth unitization treatment, based on the geological parameters of each unit fragment and tunnel parameters, the establishment of geological condition quantification matrix, combined with the corresponding excavation parameters to generate training data, and based on the training data to establish a convolutional neural network model; to obtain the geological condition quantification matrix of the section to be constructed, input the convolutional neural network model to obtain the prediction value of the corresponding tunneling parameters. In the above method, the model parameters of the convolutional neural network model can be updated after the convolutional neural network model is built to multiple sections to be constructed, or the model parame...
PUM
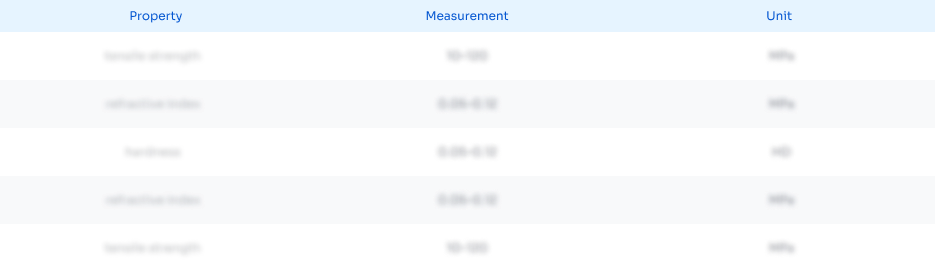
Abstract
Description
Claims
Application Information
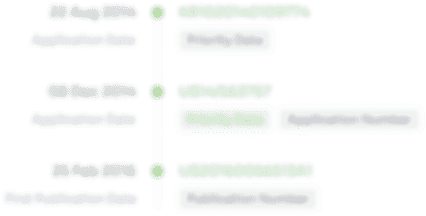
- R&D Engineer
- R&D Manager
- IP Professional
- Industry Leading Data Capabilities
- Powerful AI technology
- Patent DNA Extraction
Browse by: Latest US Patents, China's latest patents, Technical Efficacy Thesaurus, Application Domain, Technology Topic, Popular Technical Reports.
© 2024 PatSnap. All rights reserved.Legal|Privacy policy|Modern Slavery Act Transparency Statement|Sitemap|About US| Contact US: help@patsnap.com