Convolutional neural network deep learning-based environmental corrosion severity recognition method
A convolutional neural network and deep learning technology, applied in the field of testing and evaluation of electrical service environment, can solve the problems of high cost and low efficiency, and achieve the effect of reducing cost and shortening recognition time.
- Summary
- Abstract
- Description
- Claims
- Application Information
AI Technical Summary
Problems solved by technology
Method used
Image
Examples
Embodiment 1
[0048] The present invention discloses a method based on convolutional neural network depth learning environmental corrosion, which is characterized in that:
[0049] Step S1, data acquisition of multiple different electrical service environments, the data acquisition method of each electrical service environment is: placing the copper test piece in the electrical service environment, and conducts copper test pieces in multiple inspection time points Detection; with a total of N groups of real test data, each set of measured data contains an electrical service environment, a measured corrosion, and placed copper test piece placed in the electrical service environment photo data and service time. However, the photo data can characterize the corrosion state of the copper test piece, the service time is placed from the copper test piece in the electrical service environment to the time point, the photo data and the service time are normalized. deal with;
[0050] Step S2, the neural ...
Embodiment approach
[0059] like figure 1 As shown in step S2, the construction of the neural network depth learning model includes:
[0060] Step S2-1, build a photo data input module: Enter the photo data of the input neural network depth learning model, input to the convolution group of the pre-training convolutional neural network, and add a flatten layer in sequence after the convolution group, first The DENSE full connect layer and the DropOut layer are outputted image feature information; wherein the number of neurons and activation functions of the first DENSE full connection layer are set to 256 and the RELU function, and the Dropout layer has a Dropout ratio of 50%. That is, that is: Image feature data stretches into one dimensional data; the first DENSE full connection layer is used to extract the global information of the photo, and the role of the DROPOUT layer is to reduce the network over fitting.
[0061]Step S2-2, build a service time input module: Enter the service time of the input ...
Embodiment 3
[0081] Based on the above-described embodiments or of the first embodiment, the third embodiment also employs the following preferred embodiments:
[0082] The normalization process of the photo data is: decoding all of the photos of the copper test piece into an RGB pixel grid, and adjusts the height and width of each photo to the same size, and the height and The width is within 400 pixels, and each pixel value (0 to 255) of the photo is removed from 255, and each pixel value is normalized to the [0, 1] interval to obtain the N. Set photo data of data on data.
PUM
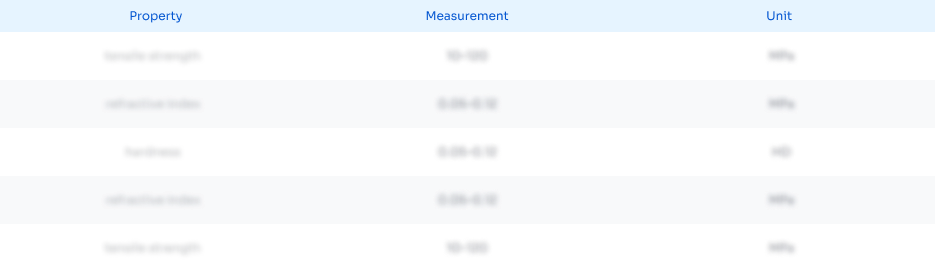
Abstract
Description
Claims
Application Information
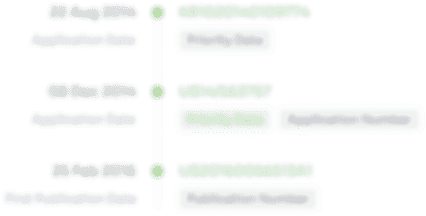
- R&D
- Intellectual Property
- Life Sciences
- Materials
- Tech Scout
- Unparalleled Data Quality
- Higher Quality Content
- 60% Fewer Hallucinations
Browse by: Latest US Patents, China's latest patents, Technical Efficacy Thesaurus, Application Domain, Technology Topic, Popular Technical Reports.
© 2025 PatSnap. All rights reserved.Legal|Privacy policy|Modern Slavery Act Transparency Statement|Sitemap|About US| Contact US: help@patsnap.com