Mechanical equipment abnormal sound detection method based on self-supervised feature extraction
A technology for mechanical equipment and feature extraction, which is applied in the testing of mechanical components, neural learning methods, testing of machine/structural components, etc., can solve the problems of low accuracy and poor correlation of abnormal sound detection of mechanical equipment, and achieve high accuracy , the effect of improving the accuracy rate
- Summary
- Abstract
- Description
- Claims
- Application Information
AI Technical Summary
Problems solved by technology
Method used
Image
Examples
Embodiment 1
[0048] Such as figure 1 with figure 2 As shown, the present invention proposes a method for detecting abnormal sound of mechanical equipment based on self-supervised feature extraction, including the following steps:
[0049] S1. Acquisition of normal operation sound data set D of mechanical equipment 1 ;
[0050] S2. Perform preprocessing and short-time Fourier transform (STFT) on all data samples to obtain normal sample time spectrum data set D 3 ;
[0051] S3. Spectrum data set D when using normal samples 2 Spectrum dataset D when abnormal samples are generated 3 ;
[0052] S4. The normal sample time spectrum data set D 2 and the generated abnormal sample time spectrum data set D 3 Combined into training data set D 4 , using D 4 Train a deep convolutional network to generate a self-supervised feature extraction model Z1;
[0053] S5. Use the self-supervised feature extraction model Z1 to extract D 2 feature T 1 , then use T 1 Train the autoencoder network to ...
Embodiment 2
[0081] Such as Figure 5 As shown, the present invention provides a mechanical equipment abnormal sound detection system based on self-supervised feature extraction, including a normal operation sound data set acquisition module, a normal sample time spectrum data set acquisition module, an abnormal sample time frequency spectrum data set acquisition module, and a self-supervised Feature extraction model generation module, abnormal detection model generation module and abnormal sound detection module;
[0082] The normal operation sound data set acquisition module is used to collect the normal operation sound data set D of mechanical equipment 1 ;
[0083] The normal sample time spectrum data set acquisition module is used to preprocess and short-time Fourier transform all data samples to obtain the normal sample time spectrum data set D 2 ;
[0084] The frequency spectrum data set acquisition module for the abnormal samples is used to use the normal sample time spectrum da...
PUM
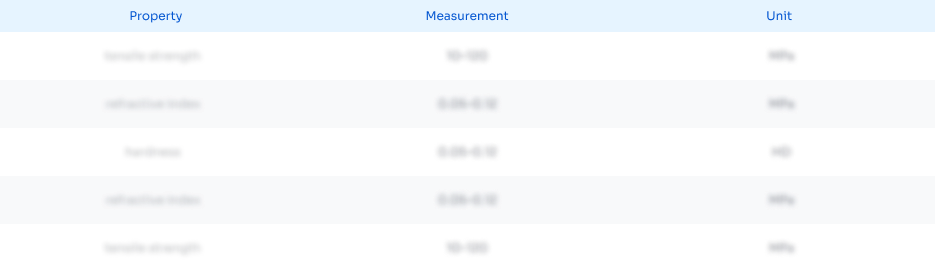
Abstract
Description
Claims
Application Information
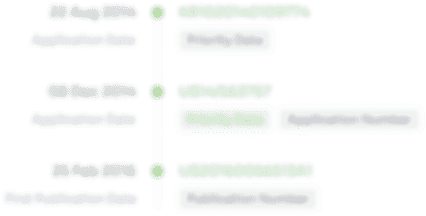
- R&D
- Intellectual Property
- Life Sciences
- Materials
- Tech Scout
- Unparalleled Data Quality
- Higher Quality Content
- 60% Fewer Hallucinations
Browse by: Latest US Patents, China's latest patents, Technical Efficacy Thesaurus, Application Domain, Technology Topic, Popular Technical Reports.
© 2025 PatSnap. All rights reserved.Legal|Privacy policy|Modern Slavery Act Transparency Statement|Sitemap|About US| Contact US: help@patsnap.com