Deep fake face data identification method
A face and data technology, which is applied in the identification field of deep fake face data, can solve the problems of image detection accuracy drop, convolution operation limited receptive field, failure to consider pixel relationship, etc., to improve robustness , Improving the effect of generalization ability
- Summary
- Abstract
- Description
- Claims
- Application Information
AI Technical Summary
Problems solved by technology
Method used
Image
Examples
Embodiment Construction
[0046] Below through specific embodiment, further describe the present invention.
[0047] Step 1: Input image X ∈ R H×W×C , where H and W are the height and width of the image, respectively, and C is the number of channels of the image (generally 3). f represents the backbone network (Efficientnet-b4[8] in the implementation of the present invention), f t is the feature map extracted from layer t. Feature maps are first extracted from the shallow layers of f
[0048] Step 2: To capture multi-scale forgery patterns, the feature map is segmented into spatial image patches of different sizes, and the self-attention between image patches of different heads is calculated. Specifically, from f s The extracted shape is r h ×r h ×C spatial image block Among them, N=(H / r h )×(W / r h ), and reshape them into 1D vectors of the hth head.
[0049]Step 3: Flatten the image patches using a fully connected layer Embedding to query embedding middle. Follow similar operations ...
PUM
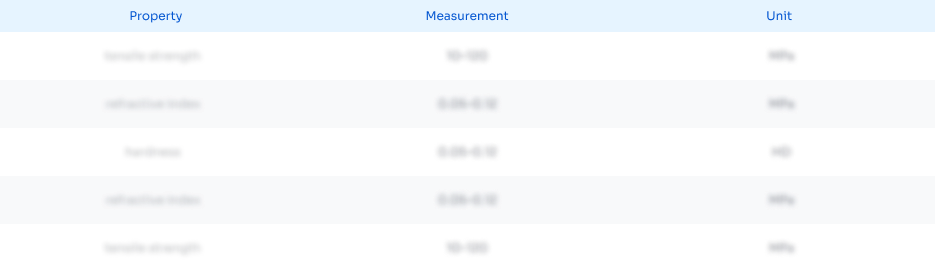
Abstract
Description
Claims
Application Information
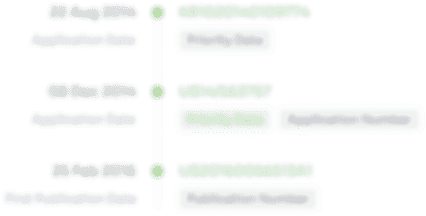
- R&D Engineer
- R&D Manager
- IP Professional
- Industry Leading Data Capabilities
- Powerful AI technology
- Patent DNA Extraction
Browse by: Latest US Patents, China's latest patents, Technical Efficacy Thesaurus, Application Domain, Technology Topic, Popular Technical Reports.
© 2024 PatSnap. All rights reserved.Legal|Privacy policy|Modern Slavery Act Transparency Statement|Sitemap|About US| Contact US: help@patsnap.com