CNN-SVM-based event-related potential signal classification method
An event-related potential and signal classification technology, applied in the direction of nuclear methods, neural learning methods, computer components, etc., can solve the problems of large individual differences, weak ERP signal signals, limiting the application of brain-computer interface engineering, etc. Application performance, reducing overfitting problems, and improving the effect of recognition accuracy
- Summary
- Abstract
- Description
- Claims
- Application Information
AI Technical Summary
Problems solved by technology
Method used
Image
Examples
Embodiment Construction
[0030] The present invention will be further described in detail below in conjunction with the embodiments and the accompanying drawings.
[0031] Such as figure 1 As shown, a CNN-SVM-based event-related potential signal classification method includes the following steps:
[0032] Step 1: Place measurement electrodes at FCz, C1, Cz, C2, Pz, and POz in the top and occipital regions of the user's head, place reference electrodes at A1 or A2 on one side of the earlobe, and place a reference electrode on the forehead of the user's head. The ground electrode is placed at the Fpz position, and the EEG signal measured by the electrode is sent to the computer after amplification and analog-to-digital conversion;
[0033] Step 2: If figure 2 As shown in Figure (a), the computer display presents a 6×6 character moment composed of 26 English letters, 9 numbers and underlines. The task of the user is to focus on the On the characters in the word, that is, one character is used at a ti...
PUM
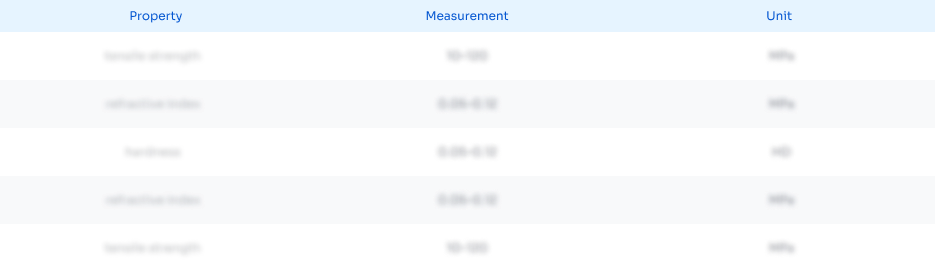
Abstract
Description
Claims
Application Information
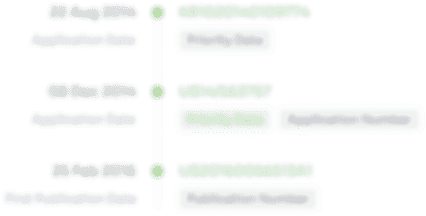
- Generate Ideas
- Intellectual Property
- Life Sciences
- Materials
- Tech Scout
- Unparalleled Data Quality
- Higher Quality Content
- 60% Fewer Hallucinations
Browse by: Latest US Patents, China's latest patents, Technical Efficacy Thesaurus, Application Domain, Technology Topic, Popular Technical Reports.
© 2025 PatSnap. All rights reserved.Legal|Privacy policy|Modern Slavery Act Transparency Statement|Sitemap|About US| Contact US: help@patsnap.com