Structural health monitoring multivariate data anomaly diagnosis method based on convolutional neural network and transfer learning
A technology of convolutional neural network and multivariate data, which is applied in the field of abnormal diagnosis of multivariate data in structural health monitoring based on convolutional neural network and transfer learning, can solve the problems of inability to achieve real-time data analysis of the monitoring system, inability to fully represent the real state of the structure, Time-consuming and labor-intensive issues
- Summary
- Abstract
- Description
- Claims
- Application Information
AI Technical Summary
Problems solved by technology
Method used
Image
Examples
specific Embodiment approach 1
[0057] Step 1: Convert the multivariate monitoring data of a large-scale structure A from time series data visualization processing to time domain response image, manually label according to the time domain response image data corresponding to the same data segment, and select various anomalies with manual labeling Types of samples form the training set D{A T ,L};
[0058] Step 2: The training set D{A T ,L} input to the convolutional neural network model CNN-A for anomaly detection;
[0059] Step 3: Visualize a small amount of multivariate monitoring data of a large structure B, and repeat step 1 to form a small training set D{B T ,L};
[0060] Step 4: Add the training set D{B on the basis of the convolutional neural network model CNN-A T ,L}, carry out migration learning training, improve the generalization performance of the classification model, and enable the convolutional neural network model to adapt to data of different distributions. The trained model is used as an...
specific Embodiment approach 2
[0082] Use pre-training model AlexNet (or other) model to replace described convolutional neural network model CNN-A, carry out migration learning on AlexNet model;
[0083] Step A: Visualize a small amount of multivariate data of structure A, and form a small data set D{A according to step 1 T ,L};
[0084] Step B: Add the training set on the basis of the AlexNet model for migration learning training, improve the generalization performance of the classification model, and enable the convolutional neural network model to adapt to data of different distributions. The trained model is used as an abnormal data detector to detect data anomalies detection;
[0085] Among them, the training ratio is 0.85, the initial learning rate is 0.0005, and the learning rate of the frozen layer is 0. Batch processing is used during training. The batch size is 128. The objective function is the cross entropy function. stochastic gradient descent with momentum;
[0086] Only the data of struct...
specific Embodiment approach 3
[0088] Using mixed data sets for migration learning, the multivariate monitoring data of structure A and structure B are combined from time series data through data visualization and manual labeling, and according to the method described in step 1, a training set containing various multivariate data of two structures A and B is formed. D{S T ,L}, and D{S T ,L} is divided into D{S with a large amount of data 1 ,L} and D{S with less data 2 ,L};
[0089] Step S1, the data set D{S 1 , L} input to the convolutional neural network model A for abnormal detection, training the convolutional neural network model, the parameter setting is the same as the parameter setting in step 2;
[0090] Step S2, the data set D{S 2 ,L} is input to the convolutional neural network model CNN-A for anomaly detection for migration training, and the parameter settings are the same as those in step 4.
PUM
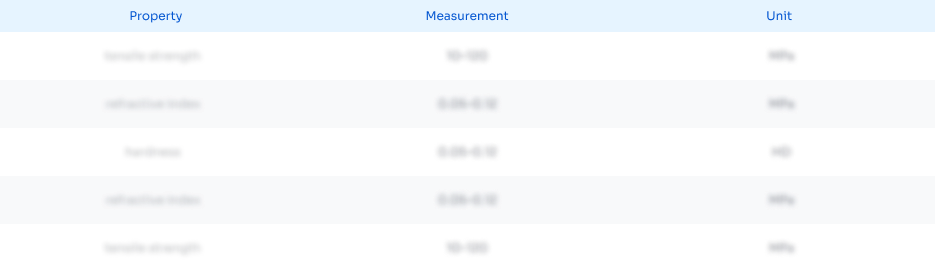
Abstract
Description
Claims
Application Information
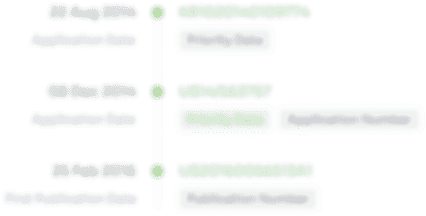
- R&D Engineer
- R&D Manager
- IP Professional
- Industry Leading Data Capabilities
- Powerful AI technology
- Patent DNA Extraction
Browse by: Latest US Patents, China's latest patents, Technical Efficacy Thesaurus, Application Domain, Technology Topic, Popular Technical Reports.
© 2024 PatSnap. All rights reserved.Legal|Privacy policy|Modern Slavery Act Transparency Statement|Sitemap|About US| Contact US: help@patsnap.com