Ultrahigh-dimensional data reconstruction deep learning method based on wavelet analysis
A technology of data reconstruction and wavelet analysis, which is applied in the fields of neural network and image processing, can solve problems such as limited video memory capacity and inability to perform deep learning training, and achieve the effect of accelerating the training process
- Summary
- Abstract
- Description
- Claims
- Application Information
AI Technical Summary
Problems solved by technology
Method used
Image
Examples
Embodiment Construction
[0044] Specific embodiments of the present invention will be described in detail below in conjunction with the accompanying drawings. It should be understood that the specific embodiments described here are only used to illustrate and explain the present invention, and are not intended to limit the present invention.
[0045] The present invention provides a multi-frequency domain parallel neural network, and creatively proposes to use wavelet packet transformation to decompose ultra-high-dimensional data (such as video data, diffusion-weighted magnetic resonance data, functional magnetic resonance data, etc.) into different frequency subbands ( subband), and build independent neural networks for each sub-domain to complete tasks such as segmentation, generation and reconstruction of high-dimensional image data. This method can be applied to deep learning tasks of large-volume ultra-high-dimensional data input such as medical image analysis and video analysis. Note: Each (wav...
PUM
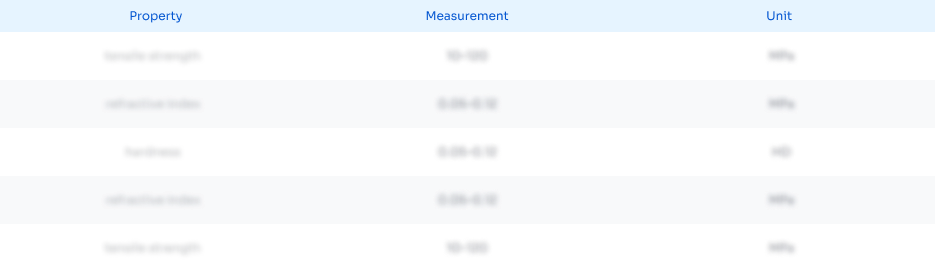
Abstract
Description
Claims
Application Information
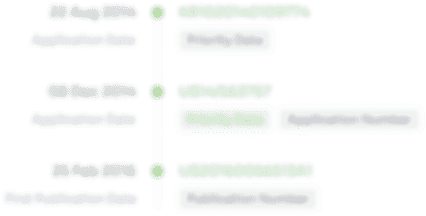
- R&D
- Intellectual Property
- Life Sciences
- Materials
- Tech Scout
- Unparalleled Data Quality
- Higher Quality Content
- 60% Fewer Hallucinations
Browse by: Latest US Patents, China's latest patents, Technical Efficacy Thesaurus, Application Domain, Technology Topic, Popular Technical Reports.
© 2025 PatSnap. All rights reserved.Legal|Privacy policy|Modern Slavery Act Transparency Statement|Sitemap|About US| Contact US: help@patsnap.com