A method for predicting the remaining life of gearbox bearings
A prediction method, gearbox technology, applied in neural learning methods, testing of mechanical components, testing of machine/structural components, etc., can solve inaccurate life prediction results, without considering other types such as current signal sensors, without consideration To achieve the effect of strengthening feature extraction and screening capabilities, avoiding deep damage, and improving prediction accuracy
- Summary
- Abstract
- Description
- Claims
- Application Information
AI Technical Summary
Problems solved by technology
Method used
Image
Examples
Embodiment Construction
[0037] Below in conjunction with embodiment, the present invention is described in further detail:
[0038] like figure 1 As shown, a method for predicting the remaining life of a gearbox bearing includes the following steps:
[0039] Step S1: Step S11, use the acceleration sensor to collect the multi-channel vibration signal of the gearbox bearing, obtain the multi-channel stator current signal from the output end of the generator through the current clamp, obtain the original multi-channel vibration signal and the multi-channel stator current signal, and carry out data preprocessing;
[0040] Step S12, performing equidistant indexing on the data in time sequence to reduce the amount of data, and the step size is M;
[0041] Step S13, perform sliding window processing to fully extract time series feature information, the window size is W, and after further normalization of the maximum and minimum values, the final data size is:
[0042] N 1 / (M*W)×1×D 1 ,N 2 / (M*W)×1×D ...
PUM
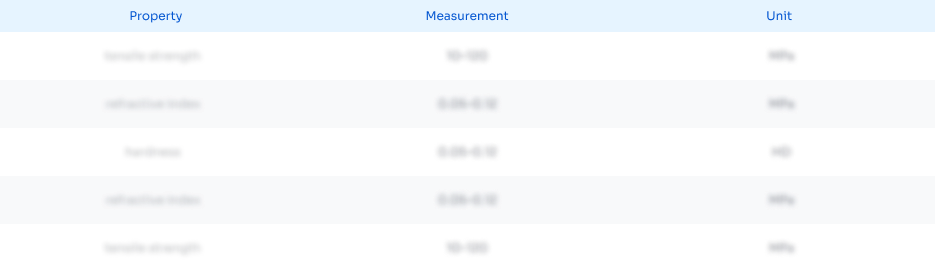
Abstract
Description
Claims
Application Information
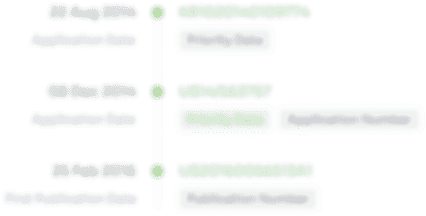
- R&D Engineer
- R&D Manager
- IP Professional
- Industry Leading Data Capabilities
- Powerful AI technology
- Patent DNA Extraction
Browse by: Latest US Patents, China's latest patents, Technical Efficacy Thesaurus, Application Domain, Technology Topic, Popular Technical Reports.
© 2024 PatSnap. All rights reserved.Legal|Privacy policy|Modern Slavery Act Transparency Statement|Sitemap|About US| Contact US: help@patsnap.com