Focus segmentation model training and application method based on semi-supervised learning
A semi-supervised learning and segmentation model technology, applied in the field of image recognition, can solve the problems of difficulty in extracting features of brain MRI images, poor effect, etc., to achieve good model training effect, reduce noise, and save costs.
- Summary
- Abstract
- Description
- Claims
- Application Information
AI Technical Summary
Problems solved by technology
Method used
Image
Examples
Embodiment 1
[0044] like figure 1 As shown, the lesion segmentation model training method based on semi-supervised learning includes steps:
[0045] S1: Build a deep learning network model in the deep learning framework, obtain all brain MRI image training samples, divide the unlabeled brain MRI images into the unlabeled training set, and divide the brain MRI images that have been correctly labeled with the lesion area label and The corresponding labels are included in the labeled training set;
[0046] The label is the lesion area in the manually marked image.
[0047] S2: Preprocessing all brain MRI image training samples;
[0048] The preprocessing includes: one or more of image format conversion, acquisition time correction, head movement correction, image fusion between different imaging methods, image registration and spatial smoothing.
[0049] S3: Input the preprocessed brain MRI image training samples into the shared encoder;
[0050] S4: The shared encoder performs multiple d...
Embodiment 2
[0077] This embodiment provides a method for applying a lesion segmentation model based on semi-supervised learning, such as figure 2 shown, including steps:
[0078] S101: input the MRI image of the brain to be segmented into the trained deep learning network model;
[0079] S102: Preprocessing the brain MRI image to be segmented;
[0080] S103: Input the preprocessed data into the shared encoder;
[0081] S104: Perform multi-domain attention mechanism processing on the results of each downsampling of the shared encoder, and input the features obtained by the last downsampling to the main decoder;
[0082] S105: The main decoder upsamples the input data. After each upsampling, the main decoder first performs feature fusion on the result of this upsampling and the output features of the multi-domain attention mechanism processing, and then performs the next step. Upsampling, the features obtained by the last upsampling are fused with the corresponding features output by th...
Embodiment 3
[0085] This embodiment provides a lesion segmentation training model based on semi-supervised learning, the model structure is as follows image 3 As shown, during the training process, the model includes: a data preprocessing module, a shared encoder, a multi-domain attention mechanism module, a main decoder, a data interference module, an auxiliary decoder, and a calculation loss module.
[0086] Brain MRI image training samples include unlabeled brain MRI images and labeled brain MRI images, divide the unlabeled brain MRI images into the unlabeled training set, and divide the brain MRI images that have been correctly labeled with the lesion area label And the corresponding labels are classified into the labeled training set;
[0087] After the model receives the input training samples, it first inputs the training samples into the data preprocessing module, and the data preprocessing module preprocesses the input data; then the preprocessed data is input into the shared enc...
PUM
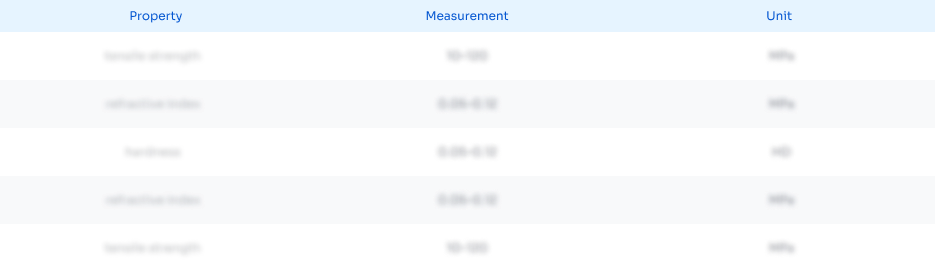
Abstract
Description
Claims
Application Information
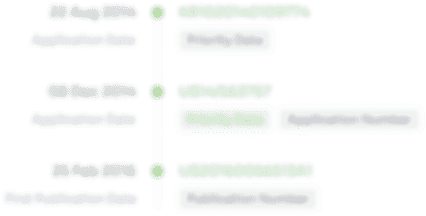
- R&D Engineer
- R&D Manager
- IP Professional
- Industry Leading Data Capabilities
- Powerful AI technology
- Patent DNA Extraction
Browse by: Latest US Patents, China's latest patents, Technical Efficacy Thesaurus, Application Domain, Technology Topic, Popular Technical Reports.
© 2024 PatSnap. All rights reserved.Legal|Privacy policy|Modern Slavery Act Transparency Statement|Sitemap|About US| Contact US: help@patsnap.com