An EEG Signal Classification Method Based on Improved Deep Residual Group Convolutional Network
An EEG signal and convolutional network technology, applied in the field of pattern recognition, can solve problems such as inability to utilize EEG signals, optimize the amount of calculation and feature transfer, and achieve the effect of improving the gradient explosion problem, easy optimization, and speeding up the convergence speed.
- Summary
- Abstract
- Description
- Claims
- Application Information
AI Technical Summary
Problems solved by technology
Method used
Image
Examples
Embodiment Construction
[0052] The present invention is described in detail below in conjunction with the accompanying drawings.
[0056] 2) The brain electrical signals of the experimenter are collected, and the collection frequency is set to 250Hz.
[0064] Using the wavelet transform to extract the EEG signal in the frequency range of 0.5Hz to 30Hz, the 1.5-dimensional EEG signal of the collected EEG
[0068] 3) Configure the improved ResNeXt.
[0079] 1) Use wavelet transform to extract the EEG signal in the frequency range of 0.5Hz to 30Hz.
[0081]
[0084] (4) Establish an EEG signal classification network based on improved ResNeXt.
[0088] The number of channels for each path of each cardinality is set to 4. ResNeXt structure parameter column
[0089]
[0090]
[0091] The activation function of the present invention selects the ReLU activation function, which can effectively improve the gradient
[0094] where x represents the output signal of a node in the ResNeXt classification network.
[00...
PUM
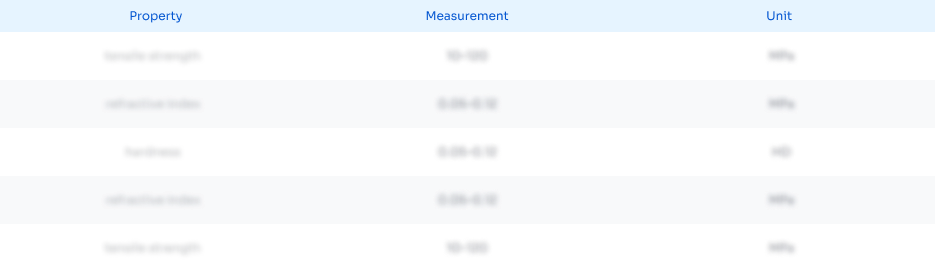
Abstract
Description
Claims
Application Information
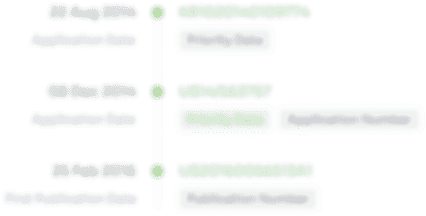
- R&D Engineer
- R&D Manager
- IP Professional
- Industry Leading Data Capabilities
- Powerful AI technology
- Patent DNA Extraction
Browse by: Latest US Patents, China's latest patents, Technical Efficacy Thesaurus, Application Domain, Technology Topic.
© 2024 PatSnap. All rights reserved.Legal|Privacy policy|Modern Slavery Act Transparency Statement|Sitemap