A deep learning method with stable performance
A deep learning and stable performance technology, applied in the field of deep learning, can solve the problems of accuracy rate drop and achieve the effect of stabilizing the test accuracy rate
- Summary
- Abstract
- Description
- Claims
- Application Information
AI Technical Summary
Problems solved by technology
Method used
Image
Examples
Embodiment Construction
[0027] The present invention proposes a deep learning method with stable performance, and the present invention is further described in detail below with reference to the accompanying drawings and specific embodiments.
[0028] The present invention proposes a deep learning method with stable performance. The overall process is as follows: figure 1 shown, including the following steps:
[0029] 1) Obtain the training data set;
[0030] Obtain a labeled image dataset that can be used for classification tasks as a training dataset, and each training sample in the training dataset contains an image and a classification label corresponding to the image. The stronger the heterogeneity in the training data, the better the effect of this method.
[0031] 2) Build a deep learning network:
[0032] The deep learning network of the present invention is composed of a deep feature extractor g (using a convolutional network) and a classifier f. The input of the deep feature extractor i...
PUM
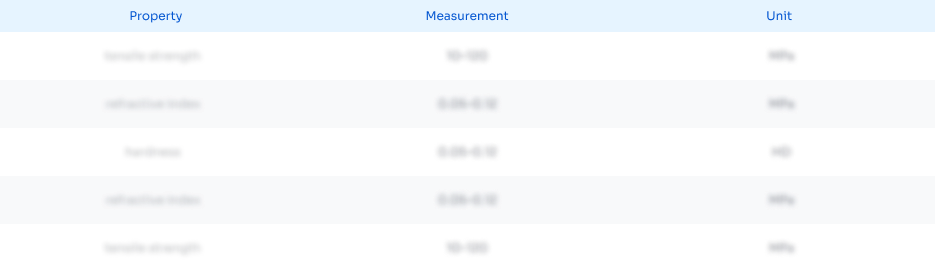
Abstract
Description
Claims
Application Information
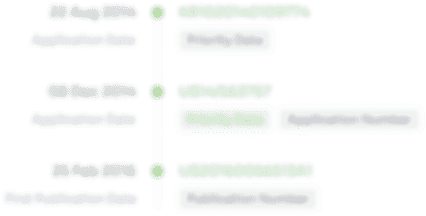
- R&D Engineer
- R&D Manager
- IP Professional
- Industry Leading Data Capabilities
- Powerful AI technology
- Patent DNA Extraction
Browse by: Latest US Patents, China's latest patents, Technical Efficacy Thesaurus, Application Domain, Technology Topic, Popular Technical Reports.
© 2024 PatSnap. All rights reserved.Legal|Privacy policy|Modern Slavery Act Transparency Statement|Sitemap|About US| Contact US: help@patsnap.com