Large-scene point cloud semantic segmentation method
A semantic segmentation and scene point technology, applied in the field of computer vision, can solve problems such as feature noise and redundancy of point cloud at the encoding layer, loss of key information, and impact on semantic segmentation performance
- Summary
- Abstract
- Description
- Claims
- Application Information
AI Technical Summary
Problems solved by technology
Method used
Image
Examples
Embodiment Construction
[0063] In order to facilitate the understanding of those skilled in the art, the present invention will be further described below in conjunction with the embodiments and accompanying drawings, and the contents mentioned in the embodiments are not intended to limit the present invention.
[0064] refer to figure 1 , Figure 7 As shown, a large scene point cloud semantic segmentation method includes the following steps:
[0065] S10: Perform feature stitching on the 3D point cloud data containing feature information to obtain the initial features of the point cloud
[0066] The feature information of the 3D point cloud data mainly includes 3D coordinate information and RGB color information. First, the feature information of the 3D point cloud data is spliced to obtain the splicing features, and then the splicing features are fused through the convolutional layer or the fully connected layer to obtain the preset Initial features of the point cloud in the output dimension. ...
PUM
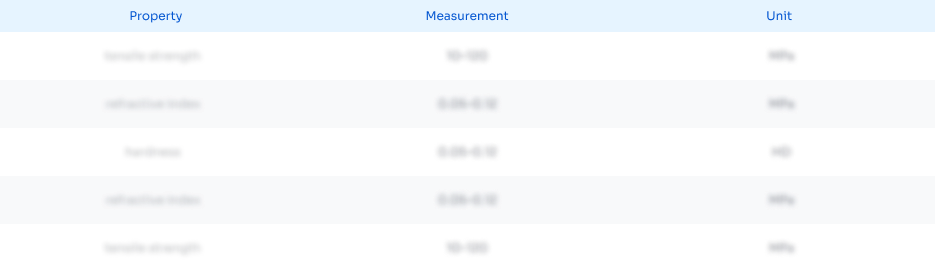
Abstract
Description
Claims
Application Information
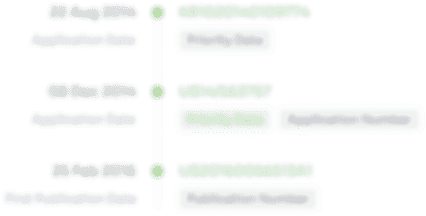
- R&D Engineer
- R&D Manager
- IP Professional
- Industry Leading Data Capabilities
- Powerful AI technology
- Patent DNA Extraction
Browse by: Latest US Patents, China's latest patents, Technical Efficacy Thesaurus, Application Domain, Technology Topic, Popular Technical Reports.
© 2024 PatSnap. All rights reserved.Legal|Privacy policy|Modern Slavery Act Transparency Statement|Sitemap|About US| Contact US: help@patsnap.com