Adaptive extraction and diagnosis method for degree features of mechanical fault through stack-type sparse automatic coding depth neural network
A sparse auto-encoding and deep neural network technology, applied in neural learning methods, biological neural network models, mechanical bearing testing, etc., can solve problems such as poor learning effect and limited learning ability
- Summary
- Abstract
- Description
- Claims
- Application Information
AI Technical Summary
Problems solved by technology
Method used
Image
Examples
Embodiment
[0096] In this embodiment, the validity of the present invention is verified by the following steps:
[0097] Step 1: Receive the vibration signals of multiple sets of rolling bearings under 9 fault degrees to be analyzed. The fault types are shown in Table 1. The bearing model is SKF6205-RS, and the damage is simulated on the inner and outer raceways and rolling elements by EDM grooves. The groove depth is 0.279mm, and the groove width represents the different damage degrees of the bearing. In Table 1, taking inner ring damage as an example, the codes IF1 / IF2 / IF3 refer to the degree of damage when the groove width is 0.178 / 0.356 / 0.533 (mm), and the larger the groove width, the more serious the bearing damage.
[0098] Table 1 Fault type
[0099]
[0100] Vibration data were collected under two working conditions of load 2HP, speed 1750rpm and load 0HP, speed 1797rpm. The sampling frequency was 48kHz, and the sampling length was 485643 points, with 2048 points as a segment...
PUM
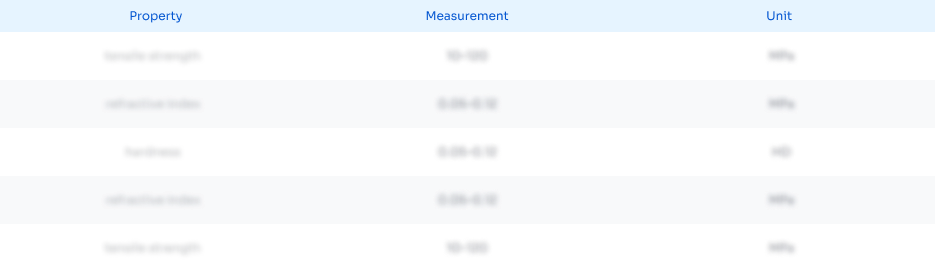
Abstract
Description
Claims
Application Information
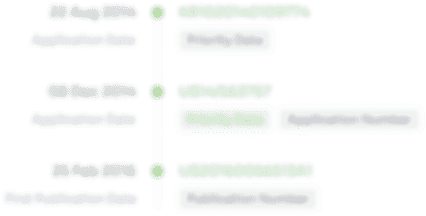
- Generate Ideas
- Intellectual Property
- Life Sciences
- Materials
- Tech Scout
- Unparalleled Data Quality
- Higher Quality Content
- 60% Fewer Hallucinations
Browse by: Latest US Patents, China's latest patents, Technical Efficacy Thesaurus, Application Domain, Technology Topic, Popular Technical Reports.
© 2025 PatSnap. All rights reserved.Legal|Privacy policy|Modern Slavery Act Transparency Statement|Sitemap|About US| Contact US: help@patsnap.com