Vehicle convergence control method based on deep reinforcement learning algorithm
A technology for strengthening learning and control methods, applied in the field of automobile driving control, can solve problems such as unfavorable driving safety, convergence failure, safety hazards, etc., and achieve the effect of improving interpretability, simplifying training difficulty, and improving safety.
- Summary
- Abstract
- Description
- Claims
- Application Information
AI Technical Summary
Problems solved by technology
Method used
Image
Examples
Embodiment Construction
[0020] Embodiments of the present invention will be described below in conjunction with the accompanying drawings.
[0021] Such as figure 2 As shown, the present invention relates to a vehicle merging control method based on deep reinforcement learning algorithm, the method mainly includes the following steps:
[0022] Step 1. Decompose the vehicle merging scene into several problem points, and divide them into two types of problem points that are suitable for and unsuitable for deep reinforcement learning training, as follows:
[0023] Step 1.1: The process of decomposing the vehicle merging scene, which can be decomposed but not limited to five points: whether it is necessary to merge, whether there are other vehicles in the merging lane, whether other vehicles are merging, where to merge, and at what speed to merge.
[0024] Step 1.2: Divide the problem points, which can be judged based on past experience. If there is a better and simpler algorithm, use the corresponding...
PUM
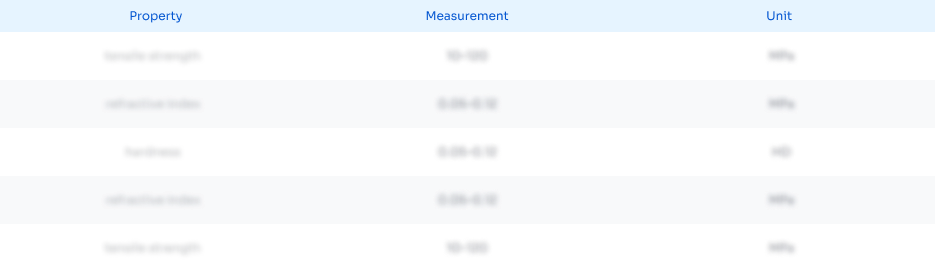
Abstract
Description
Claims
Application Information
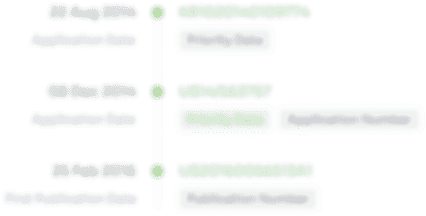
- R&D Engineer
- R&D Manager
- IP Professional
- Industry Leading Data Capabilities
- Powerful AI technology
- Patent DNA Extraction
Browse by: Latest US Patents, China's latest patents, Technical Efficacy Thesaurus, Application Domain, Technology Topic, Popular Technical Reports.
© 2024 PatSnap. All rights reserved.Legal|Privacy policy|Modern Slavery Act Transparency Statement|Sitemap|About US| Contact US: help@patsnap.com