Pedestrian multi-target tracking method combining attention mechanism end-to-end training
A multi-target tracking and attention technology, applied in the field of computer vision, can solve the problems of complex calculation process, increased calculation amount and memory overhead, etc., to achieve the effect of improving calculation efficiency, reducing calculation overhead, and improving discrimination ability.
- Summary
- Abstract
- Description
- Claims
- Application Information
AI Technical Summary
Problems solved by technology
Method used
Image
Examples
Embodiment 1
[0053] A pedestrian multi-object tracking method trained end-to-end with a joint attention mechanism, such as figure 1 As shown, it includes the following steps: Step S100: Collect a pedestrian data set of labeled video sequences, and use the first frame of the real bounding box of each video in the label to initialize the tracking box as a template sample, and then according to the center of the tracking box in Cut out the positive search area sample in the second frame, and cut out the negative search area sample in the area that is not the same target; The template sample, the positive search area sample, and the negative search area sample form a triplet, which is output as a training sample;
[0054] Step S200: Construct a deep neural network model, use the convolutional neural network to partially extract the feature information of the sample, and then use the attention mechanism module to guide the network model to tend to important feature information, and finally calcu...
Embodiment 2
[0059] This embodiment is optimized on the basis of embodiment 1, such as figure 1 , figure 2 As shown, the step S200 includes the following steps:
[0060] Step S201: Construct three network structure branches respectively processing template samples, positive search area samples, template sample branches of negative search area samples, positive search area sample branches, and negative search sample branches, the template sample branch, positive search area samples The backbone network structures of branches and negative search sample branches are the same, and share weight parameters;
[0061] Step S202: Both the positive search region sample branch and the negative search sample branch use the region-of-interest alignment layer downsampling feature point information, and the backbone network of the positive search region sample branch and the negative search sample branch is connected to the region-of-interest alignment layer An attention mechanism module is set betwee...
Embodiment 3
[0071] This embodiment is optimized on the basis of Embodiment 1 or 2. The verification loss function in step S300 adopts a flexible maximum loss function, and the calculation formula is as follows:
[0072]
[0073] where: z i 、x i 、x j Respectively represent template samples, positive search area samples, and negative search area samples;
[0074] Respectively represent the predicted probability value of the template sample, the predicted probability value of the positive search area sample, and the predicted probability value of the negative search area sample;
[0075] Increase the classification ability of the model by minimizing the validation loss function;
[0076] The single target tracking loss function is calculated by convolving the heat map obtained from the feature map output by the backbone network part. The calculation formula is as follows:
[0077]
[0078] Where: p is a feature point on the heat map,
[0079] P is the feature map,
[0080] v p...
PUM
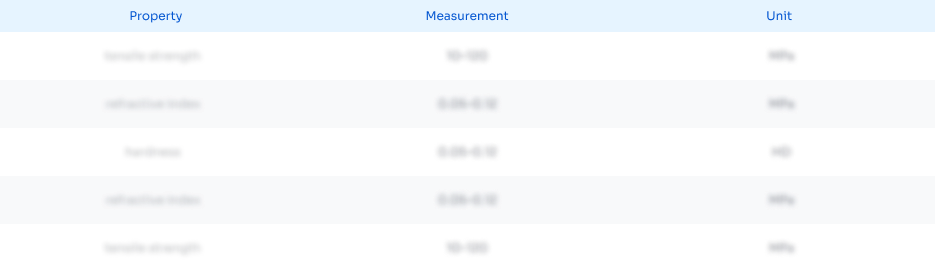
Abstract
Description
Claims
Application Information
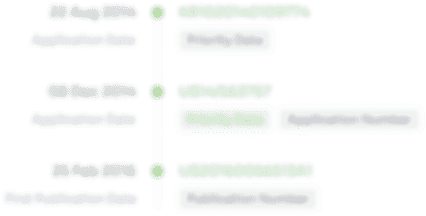
- R&D Engineer
- R&D Manager
- IP Professional
- Industry Leading Data Capabilities
- Powerful AI technology
- Patent DNA Extraction
Browse by: Latest US Patents, China's latest patents, Technical Efficacy Thesaurus, Application Domain, Technology Topic, Popular Technical Reports.
© 2024 PatSnap. All rights reserved.Legal|Privacy policy|Modern Slavery Act Transparency Statement|Sitemap|About US| Contact US: help@patsnap.com