Intelligent QoS routing optimization method and system based on deep reinforcement learning in SDN environment
A technology of reinforcement learning and optimization methods, applied in the field of network routing optimization, can solve problems such as difficulty in coping with the network environment and high computational overhead
- Summary
- Abstract
- Description
- Claims
- Application Information
AI Technical Summary
Problems solved by technology
Method used
Image
Examples
Embodiment
[0126] Experimental environment: the SDN controller in this embodiment uses Floodlight1.2, the network topology is constructed using the network emulator Mininet2.3, and the Python program is used to realize the agent of deep reinforcement learning, and the Iperf tool is selected to simulate the transmission of network services.
[0127] Network topology: such as Figure 9 As shown, the real NSFNET network is deployed in the Mininet network simulation software, which contains 13 switch nodes and 20 links. Among them, node 0 is used as the source node, connected to the video server, and 8, 9, 11, 12, 13 are used as 5 Nodes connected to clients, 2, 4, and 7 are congested nodes.
[0128] Experimental parameters: The server (server) is responsible for sending video traffic, the video bit rate is set to 1Mbps, and the maximum delay and jitter allowed during transmission are set to 150ms and 30ms respectively. In deep reinforcement learning, the neural network parameters are set as...
PUM
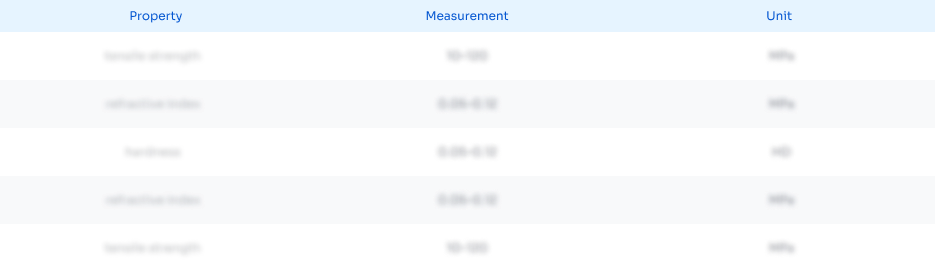
Abstract
Description
Claims
Application Information
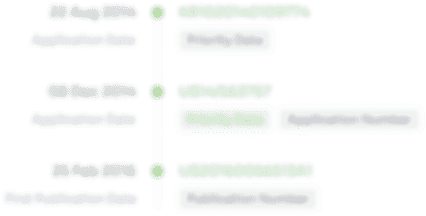
- R&D Engineer
- R&D Manager
- IP Professional
- Industry Leading Data Capabilities
- Powerful AI technology
- Patent DNA Extraction
Browse by: Latest US Patents, China's latest patents, Technical Efficacy Thesaurus, Application Domain, Technology Topic, Popular Technical Reports.
© 2024 PatSnap. All rights reserved.Legal|Privacy policy|Modern Slavery Act Transparency Statement|Sitemap|About US| Contact US: help@patsnap.com