Medical image segmentation method based on deep learning
A medical image and deep learning technology, applied in image analysis, image enhancement, image data processing, etc., can solve problems such as inability to extract finer semantic features, susceptible to noise interference, single convolution kernel scale, etc., to achieve image effects The effect of coherence, strong resistance to noise interference, and strong generalization ability
- Summary
- Abstract
- Description
- Claims
- Application Information
AI Technical Summary
Problems solved by technology
Method used
Image
Examples
Embodiment 1
[0023] The specific process of realizing medical image segmentation in this embodiment is as follows:
[0024] (2) Obtain medical images, the number of which requires more than 15. Each medical image is equipped with a segmentation mask, which is used as the label image used in model training, and the original medical image and label image are preprocessed and adjusted. resolution so that the image has a width of 256 and a height of 192;
[0025](2) Build a multi-scale semantic convolution module MS Block. The multi-scale semantic convolution module MS Block contains four branches. The first branch is a 3x3 convolution, and the second branch is two consecutive 3x3 convolutions. To replace a 5x5 convolution to achieve the same receptive field, the third branch has three 3x3 convolutions, which are the same as the receptive field of the 7x7 convolution kernel. The first, second, and third branches each have one The residual edge with 1x1 convolution is used to make up for part ...
Embodiment 2
[0031] This embodiment adopts the technical solution of Embodiment 1, and uses Keras as the deep learning framework. The experimental environment is Ubuntu 18.04, NVIDIA RTX 2080Ti (12GB, 1.545GHZ) GPU, the number of network layers is 9 layers, in the first layer network between MS Block1 and MS Block9, t=4, that is, use 1x1 convolution The number of channels is expanded by 4 times. Since the semantic gap between the encoder and the decoder is the largest in the first layer of the network, the most nonlinear transformations should be added. By analogy, from the second layer to the fourth layer of the network, Set t=3, 2, 1 in turn, taking the first layer of the network structure as an example, after the feature map output from MS Block1 passes through the RB Attention structure, it is directly stitched together with the feature map sampled on MS Block8, and finally together Input into MS Block9, this embodiment is consistent with the number of channels of each layer in the exi...
PUM
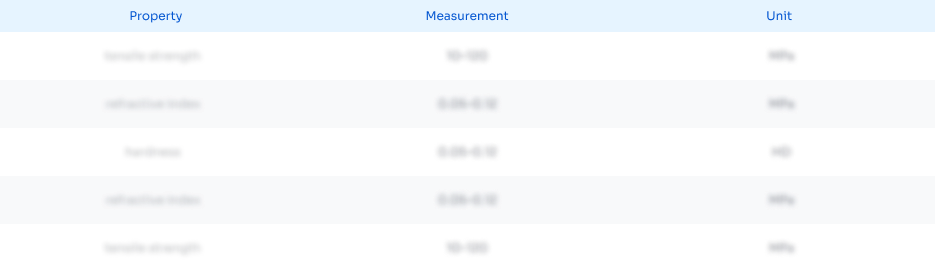
Abstract
Description
Claims
Application Information
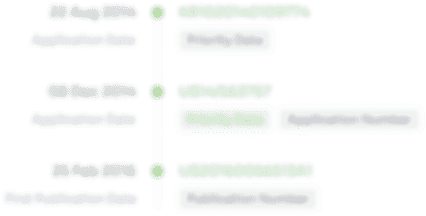
- R&D Engineer
- R&D Manager
- IP Professional
- Industry Leading Data Capabilities
- Powerful AI technology
- Patent DNA Extraction
Browse by: Latest US Patents, China's latest patents, Technical Efficacy Thesaurus, Application Domain, Technology Topic.
© 2024 PatSnap. All rights reserved.Legal|Privacy policy|Modern Slavery Act Transparency Statement|Sitemap