Sonar image library construction method based on modified CycleGAN model
A construction method and image library technology, applied in the field of sonar image library construction, can solve the problems of inability to collect sonar images, difficult to obtain data samples, and difficult operations, so as to alleviate mode collapse, increase stability, and speed up convergence. effect of speed
- Summary
- Abstract
- Description
- Claims
- Application Information
AI Technical Summary
Problems solved by technology
Method used
Image
Examples
Embodiment 1
[0042] Based on the improved CycleGAN implementation of the sonar image library method, through continuous training and optimization of the network, the mapping relationship between optics and sonar images is learned, and the corresponding sonar images can be directly synthesized from optical images by using the mapping relationship. The CycleGAN model training flow chart is as follows figure 2 As shown, the steps are as follows:
[0043] Step 1, prepare the optical image dataset and the sonar image dataset, and place them in the source domain (X domain) and the target domain (Y domain) respectively.
[0044] Step 2, set initialization parameters, including initial learning rate, batch size (ie, Batchsize value), choice of optimizer, and hyperparameters used to constrain the proportion of each loss function. The specific parameter settings are shown in Table 1.
[0045] Table 1
[0046] parameter size setting optimizer Adam initial learning rate 2e-4...
Embodiment 2
[0062] The present invention is to the improvement of CycleGAN model, and concrete improvement is divided into following several steps:
[0063] The CycleGAN network does not require a matching data set when implementing image style transfer, and has certain advantages in texture and color conversion. However, CycleGAN is prone to model collapse during the training process. Next, improve the CycleGAN loss function to Mitigating this from happening makes the final composited sonar image look better.
[0064] In step a, the log likelihood loss in the original GAN loss formula is replaced by square loss, which can increase the stability of network training. The corresponding square loss function expression is as follows:
[0065]
[0066] Among them, X and Y represent the source domain (optical image domain) and target domain (sonar image domain); G and D represent the generator and discriminator respectively; G(x): X → Y, represents the generation from the optical image So...
Embodiment 3
[0080] According to CycleGAN to realize the idea of image style transfer, its main task is to learn the mapping relationship between optical images and sonar images, and obtain the best possible mapping relationship through loss function and optimization algorithm. The specific experimental steps are as follows:
[0081] Step A, data set acquisition, that is, optical images and sonar images of different targets were collected respectively, and used to train the CycleGAN model. The present invention carries out synthesis experiments on the sonar images of three objects, which are respectively triangles, beverage bottles and tires. Therefore, corresponding optical and sonar images need to be acquired, and each experiment is performed independently. For optical images, since there is no ready-made modem available, they are obtained through web crawling (such as beverage bottles, tires) and manual drawing using drawing software (such as tripods). In this embodiment, the Gemini7...
PUM
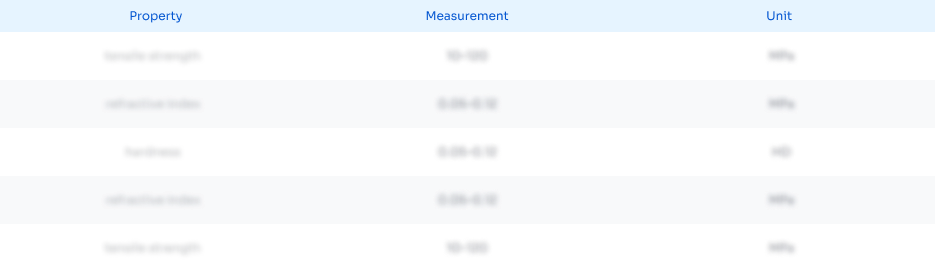
Abstract
Description
Claims
Application Information
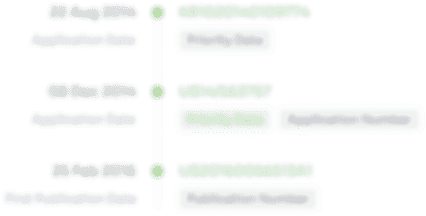
- R&D Engineer
- R&D Manager
- IP Professional
- Industry Leading Data Capabilities
- Powerful AI technology
- Patent DNA Extraction
Browse by: Latest US Patents, China's latest patents, Technical Efficacy Thesaurus, Application Domain, Technology Topic, Popular Technical Reports.
© 2024 PatSnap. All rights reserved.Legal|Privacy policy|Modern Slavery Act Transparency Statement|Sitemap|About US| Contact US: help@patsnap.com