Neoantigen identification with pan-allele models
An allele and antigen technology, applied in genomics, receptors/cell surface antigens/cell surface determinants, library member identification, etc., can solve the problems of inefficient use of autoimmunity and missing candidate neoantigens in vaccines
- Summary
- Abstract
- Description
- Claims
- Application Information
AI Technical Summary
Problems solved by technology
Method used
Image
Examples
example
[0430] In another embodiment, the deviation parameter θ h 0 May be shared by gene families according to the MHC allele h. That is, the bias parameter θ of the MHC allele h h 0 can be equal to θ 基因(h) 0 , where gene (h) is the gene family of the MHC allele h. For example, the class I MHC alleles HLA-A*02:01, HLA-A*02:02, and HLA-A*02:03 can be assigned to the "HLA-A" gene family, and these MHC alleles The respective deviation parameter θ h 0 Can be shared. As another example, the class II MHC alleles HLA-DRB1:10:01, HLA-DRB1:11:01, and HLA-DRB3:01:01 can be assigned to the "HLA-DRB" gene family, and these MHC alleles The gene's respective bias parameter θ h 0 Can be shared.
[0431] Returning to equation (2), as an example, using the affine dependency function g h (·) Of the m = 4 different MHC alleles identified, the peptide p k The probability of being presented by the MHC allele h=3 can be given by:
[0432]
[0433] where x 3 k is the allelic interaction...
Embodiment 1
[0482] VIII.C.1. Example 1: Maximum of independent allele models
[0483] In one embodiment, the training module 316 makes the peptide p associated with a set of multiple MHC alleles H k The estimated probability of presentation u k Presentation probability u of each MHC allele h in pool H as determined based on cells expressing the monoallele k h∈H Variation modeling of , as described above in conjunction with equations (2)-(10). Precisely, the presentation probability u k can be u k h∈H of any function. In one embodiment, as shown in equations (11), (12a) and (12b), the function is a maximum function and presents the likelihood u k It can be determined as the maximum likelihood of presentation for each MHC allele h in set H.
[0484]
[0485] VIII.C.2. Example 2.1: Funciton-of-Sums model
[0486] In one embodiment, the training module 316 makes the peptide p by the formula k The estimated probability of presentation u k Modeling:
[0487]
[0488] where...
Embodiment 2
[0498] VIII.C.3. Example 2.2: Functional model using sums of allelic non-interacting variables
[0499] In one embodiment, the training module 316 incorporates allelic non-interacting variables and makes the peptide p by k The estimated probability of presentation u k Modeling:
[0500]
[0501] where w k represents the encoded related peptide p k Allelic non-interacting variables. Specifically, the set of parameters θ for each MHC allele h h and the set of parameters θ about the allele non-interacting variables w The value of θ can be obtained by making h and θ w is determined by minimizing the loss function of , where i is each instance in the subset S of training data 170 generated by cells expressing a single MHC allele and / or cells expressing multiple MHC alleles. Dependency function g w can take the form of the dependency function g described in section VIII.B.3 above w any of the forms.
[0502] Therefore, according to equation (14), the function g can b...
PUM
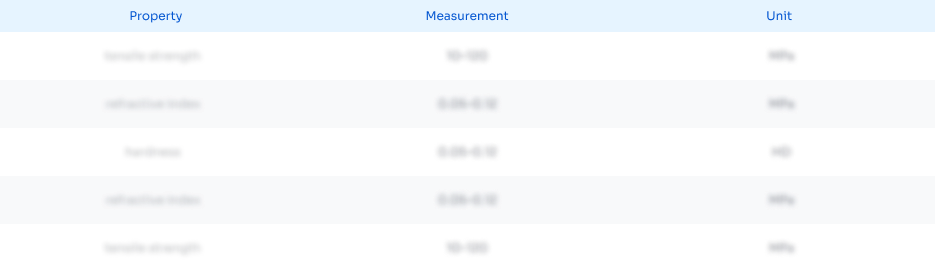
Abstract
Description
Claims
Application Information
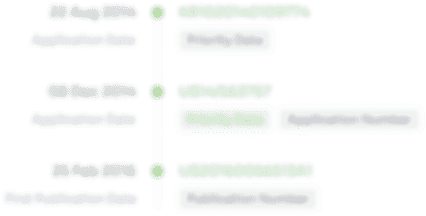
- R&D Engineer
- R&D Manager
- IP Professional
- Industry Leading Data Capabilities
- Powerful AI technology
- Patent DNA Extraction
Browse by: Latest US Patents, China's latest patents, Technical Efficacy Thesaurus, Application Domain, Technology Topic, Popular Technical Reports.
© 2024 PatSnap. All rights reserved.Legal|Privacy policy|Modern Slavery Act Transparency Statement|Sitemap|About US| Contact US: help@patsnap.com