Complex electromechanical system abnormal state detection method based on multi-source data
Anomaly detection, electromechanical system technology, applied in data processing applications, neural learning methods, resources, etc., can solve the problem of not considering complex coupling relationships, low sensitivity to system operating states, and reliability of calculation results that are difficult to meet industrial time series data anomaly detection. and other problems to achieve the effect of improving robustness and accuracy and overcoming experience dependence.
- Summary
- Abstract
- Description
- Claims
- Application Information
AI Technical Summary
Problems solved by technology
Method used
Image
Examples
Embodiment 1
[0045] This embodiment provides a complex electromechanical system anomaly detection method based on multi-source data, including the following steps:
[0046] (1) Obtain the multi-source state variables of electromechanical equipment collected by the data acquisition system, the data including temperature, pressure, and vibration data;
[0047] (2) Using non-stationary nonlinear inter-sequence correlation analysis methods (including detrended cross-correlation analysis (DCCA) method, detrended covariance function analysis method, Pearson correlation coefficient method) Quantitative analysis of the coupling relationship of the system is carried out, and the multivariate coupling relationship matrix of the system is obtained;
[0048] (3) if figure 2 As shown, the multi-source variables of the system are abstracted as nodes of the network, and the coupling relationship between two of the multi-source variables is abstracted as the edges of the network, thereby constructing a ...
Embodiment 2
[0075] Below in conjunction with the abnormal state detection of the steam turbine rotor system, the present invention will be further described:
PUM
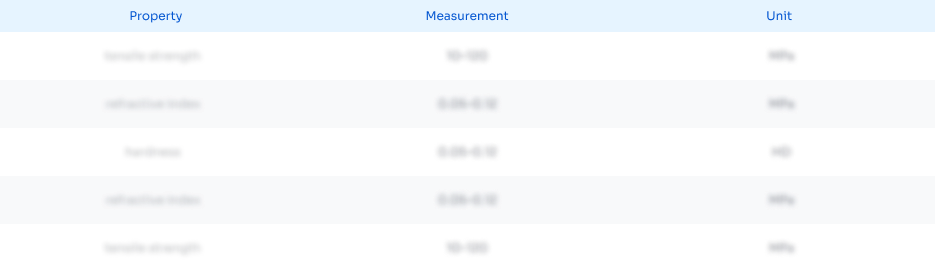
Abstract
Description
Claims
Application Information
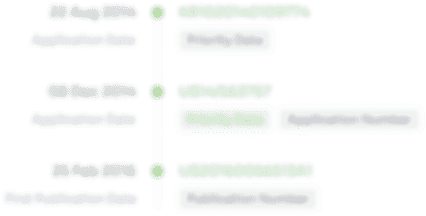
- R&D Engineer
- R&D Manager
- IP Professional
- Industry Leading Data Capabilities
- Powerful AI technology
- Patent DNA Extraction
Browse by: Latest US Patents, China's latest patents, Technical Efficacy Thesaurus, Application Domain, Technology Topic, Popular Technical Reports.
© 2024 PatSnap. All rights reserved.Legal|Privacy policy|Modern Slavery Act Transparency Statement|Sitemap|About US| Contact US: help@patsnap.com