Deep convolutional neural network automatic horizon tracking method based on constant fast mapping
A technology of convolutional neural network and layer tracking, which is applied in the field of automatic layer tracking of deep convolutional neural network, can solve the problems of self-organizing map network initialization, good effect, multiple training data, etc.
- Summary
- Abstract
- Description
- Claims
- Application Information
AI Technical Summary
Problems solved by technology
Method used
Image
Examples
Embodiment Construction
[0048] Technical principle relevant to the present invention:
[0049] convolutional neural network
[0050] A convolutional neural network is a special type of deep feed-forward neural network that consists of neurons with weights and biases. By adopting the method of partial connection, the redundancy problem caused by full connection is effectively avoided, and the parameter amount of the network is effectively reduced, thereby reducing the dependence of the model on the data itself. For example, each neuron is only connected to 10×10 pixel values. Neural network connection methods such as figure 1 As shown, (a) is the original connection (full connection), (b) is the new connection method (partial connection):
[0051] A convolutional neural network generally consists of a convolutional layer, a Relu nonlinear activation layer, a pooling layer, and a fully connected layer. Among them, you can choose whether to use the pooling layer according to the task itself. The def...
PUM
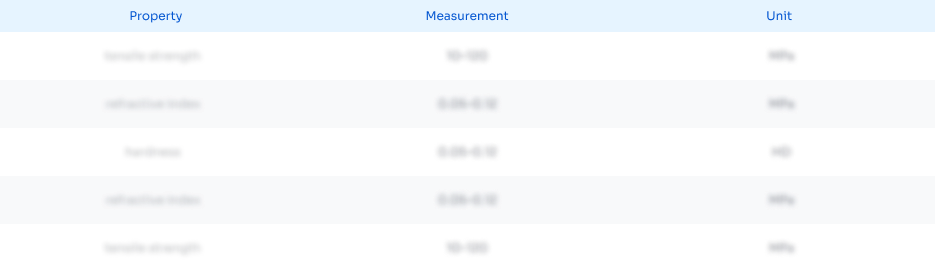
Abstract
Description
Claims
Application Information
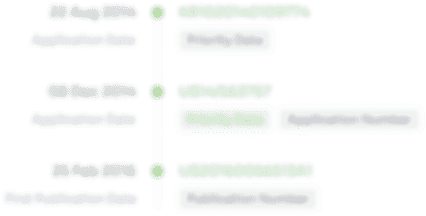
- R&D Engineer
- R&D Manager
- IP Professional
- Industry Leading Data Capabilities
- Powerful AI technology
- Patent DNA Extraction
Browse by: Latest US Patents, China's latest patents, Technical Efficacy Thesaurus, Application Domain, Technology Topic, Popular Technical Reports.
© 2024 PatSnap. All rights reserved.Legal|Privacy policy|Modern Slavery Act Transparency Statement|Sitemap|About US| Contact US: help@patsnap.com