Secondary radar signal processing method based on two-channel residual error deep neural network
A technology of deep neural network and secondary radar, which is applied in the field of secondary radar signal processing based on two-channel residual deep neural network, can solve the problem of affecting signal transmission clarity, reduction of radio wave transmission stability and reliability, noise residue, etc. problem, to achieve excellent denoising performance, reduce information loss, and meet the needs of noise suppression
- Summary
- Abstract
- Description
- Claims
- Application Information
AI Technical Summary
Problems solved by technology
Method used
Image
Examples
Embodiment
[0020] This example includes the following steps:
[0021] The first step is to obtain a sample data set: the secondary radar response timing signal with a total number of samples of 60,000 and a time step of 512 is used as sample data. The secondary radar response timing signal after adding Gaussian white noise SNR=5 and demodulation is used as the training data set, denoted as Among them, N=60000 represents the number of signal samples, and Z=512 represents the signal time step. The original secondary radar response signal without adding noise is used as the training label, denoted as And the sample data is divided into training set, verification set and test set according to the ratio of (0.6,0.2,0.2).
[0022] The second step is to preprocess the data set: randomly scramble the secondary radar training sample data, expand the dimension of the sample data and labels, and form a 3D tensor of the form (n, t, g), where n represents The number of samples, t represents the ...
PUM
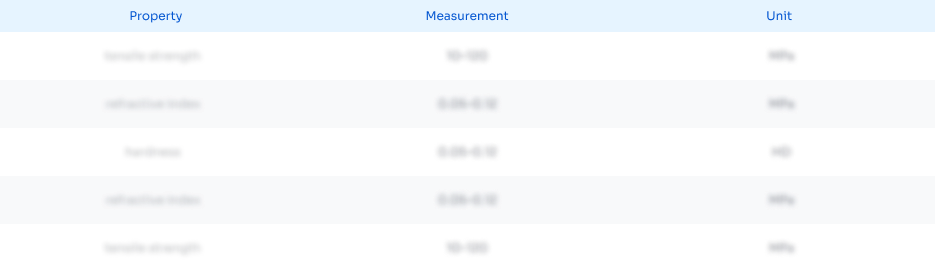
Abstract
Description
Claims
Application Information
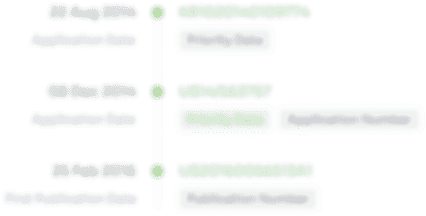
- R&D Engineer
- R&D Manager
- IP Professional
- Industry Leading Data Capabilities
- Powerful AI technology
- Patent DNA Extraction
Browse by: Latest US Patents, China's latest patents, Technical Efficacy Thesaurus, Application Domain, Technology Topic, Popular Technical Reports.
© 2024 PatSnap. All rights reserved.Legal|Privacy policy|Modern Slavery Act Transparency Statement|Sitemap|About US| Contact US: help@patsnap.com