Multi-task relationship learning method for centrum positioning, identification and segmentation in nuclear magnetic resonance imaging
A technology of nuclear magnetic resonance image and learning method, applied in the field of medical image processing, can solve the problem of ignoring the close connection between tasks
- Summary
- Abstract
- Description
- Claims
- Application Information
AI Technical Summary
Problems solved by technology
Method used
Image
Examples
Embodiment 1
[0107] A multi-task relational learning method for vertebral body localization, recognition and segmentation in MRI images, such as figure 1 shown, including the following steps:
[0108] (1) Image preprocessing
[0109] By preprocessing the NMR images and semantic segmentation labels, the final data structure meets the requirements for input and loss function calculation of the multi-task relational learning network model; in this embodiment, the NMR images refer to MR lumbar spine images ;
[0110] (2) Build a multi-task relational learning network model
[0111] like figure 2 As shown, the multi-task relational learning network model includes Seg-Loc network, XOR operation and discriminant network;
[0112] As a generator for adversarial training, the Seg-Loc network uses the task mutual attention module to learn the relationship between semantic localization and semantic segmentation end-to-end through network parameter learning, and outputs semantic localization resu...
Embodiment 2
[0127] According to a kind of multi-task relational learning method that is used for the vertebral body location, identification and segmentation in the magnetic resonance image described in embodiment 1, its difference is:
[0128] In step (1), comprise steps as follows:
[0129] The original MRI image faces some challenges, such as the weak edge information of the vertebral body; the strong noise leads to uneven gray scale of the vertebral body imaging; the diversity of resolution leads to different sizes of vertebrae in the data set; and the generated MRI spine image contains With different degrees of lesions, each image contains different numbers of vertebral bodies. After statistics, the number of MRI images containing 6 vertebral bodies (S1-L5), 7 vertebral bodies (S1-T12), and 8 vertebral bodies (S1-T11) are nearly equal.
[0130] A. First adjust all MRI images to 512*512;
[0131] B. Use ITK-SNAP software to label all MRI images with vertebral body segmentation label...
Embodiment 3
[0141] According to a kind of multi-task relational learning method that is used for the vertebra body location, identification and segmentation in the nuclear magnetic resonance image described in embodiment 2, its difference is:
[0142] In step (2), the Seg-Loc network is the generative network of the proposed multi-task relational learning network. like image 3 As shown, the structure of the Seg-Loc network is an encoder-decoder network. The encoder-decoder network includes an encoder, two decoders, and two task mutual attention modules. The two decoders share an encoder. There are two encoders between the two decoders. A task mutual attention module;
[0143] The two decoders output semantic positioning results and semantic segmentation results respectively; the task mutual attention module is used to learn the relationship between semantic positioning and semantic segmentation;
[0144] The encoder includes convolutional layer, LSTM, hole convolution group, batch norm...
PUM
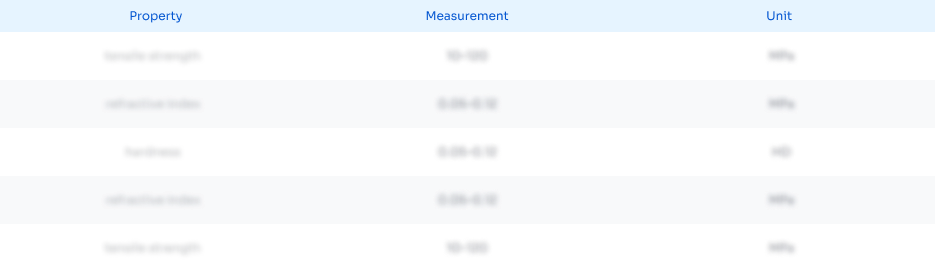
Abstract
Description
Claims
Application Information
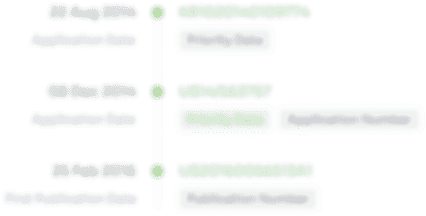
- R&D Engineer
- R&D Manager
- IP Professional
- Industry Leading Data Capabilities
- Powerful AI technology
- Patent DNA Extraction
Browse by: Latest US Patents, China's latest patents, Technical Efficacy Thesaurus, Application Domain, Technology Topic, Popular Technical Reports.
© 2024 PatSnap. All rights reserved.Legal|Privacy policy|Modern Slavery Act Transparency Statement|Sitemap|About US| Contact US: help@patsnap.com