Method for maximizing system benefits in dynamic environment based on deep reinforcement learning
A technology of reinforcement learning and dynamic environment, applied in the direction of neural learning methods, data processing applications, prediction, etc., can solve problems such as inability to provide computing services to end users
- Summary
- Abstract
- Description
- Claims
- Application Information
AI Technical Summary
Problems solved by technology
Method used
Image
Examples
Embodiment Construction
[0058] The following will further clarify the relevant content of the present invention in conjunction with the method flow chart, system model diagram, and specific algorithm framework diagram of the design in the accompanying drawings. It should be understood that these embodiments are only used to illustrate the present invention and not to limit the present invention. scope, after reading the present invention, the modifications of various equivalent forms of the present invention by those skilled in the art all fall within the scope defined by the appended claims of the present application.
[0059]The present invention focuses on the reasonable and efficient path planning and design for the UAV when the UAV is used as a mobile edge server in the edge computing architecture to provide highly reliable and low-delay computing services for terminal real-time mobile users based on the deep reinforcement learning algorithm.
[0060] As an example, the method needs to consider: ...
PUM
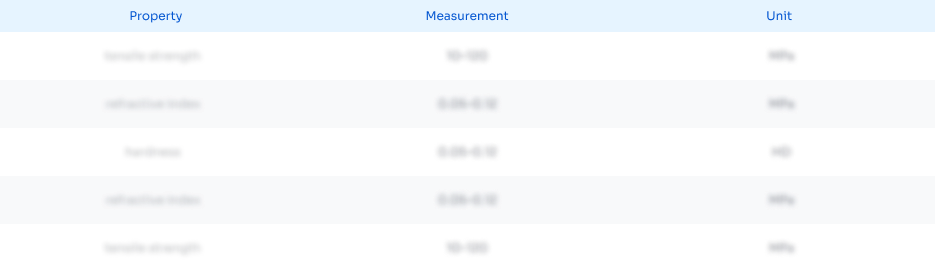
Abstract
Description
Claims
Application Information
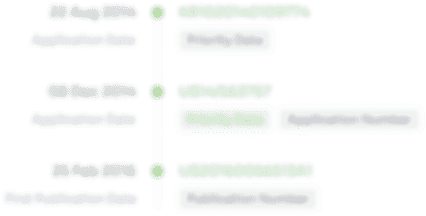
- R&D Engineer
- R&D Manager
- IP Professional
- Industry Leading Data Capabilities
- Powerful AI technology
- Patent DNA Extraction
Browse by: Latest US Patents, China's latest patents, Technical Efficacy Thesaurus, Application Domain, Technology Topic, Popular Technical Reports.
© 2024 PatSnap. All rights reserved.Legal|Privacy policy|Modern Slavery Act Transparency Statement|Sitemap|About US| Contact US: help@patsnap.com