Action recognition method for high-time-sequence 3D neural network based on cavity convolution
A neural network and action recognition technology, applied in the fields of artificial intelligence and computer vision, which can solve problems such as ignoring interaction
- Summary
- Abstract
- Description
- Claims
- Application Information
AI Technical Summary
Problems solved by technology
Method used
Image
Examples
Embodiment Construction
[0053] In order to have a clearer understanding of the technical features, purposes and effects of the present invention, the specific implementation manners of the present invention will now be described in detail with reference to the accompanying drawings.
[0054] Embodiments of the present invention provide an action recognition method based on dilated convolution-based high-sequence 3D neural network.
[0055] Please refer to figure 1 , figure 1 It is a flowchart of an action recognition method based on a high-sequence 3D neural network based on atrous convolution in an embodiment of the present invention, and specifically includes the following steps:
[0056] S101: Obtain a public data set, and divide the data set into a training set and a test set; the public data set includes two public data sets of UCF101 and HMDB51;
[0057] S102: Improving the three-dimensional Inception-V1 neural network model to obtain an improved three-dimensional Inception-V1 neural network ...
PUM
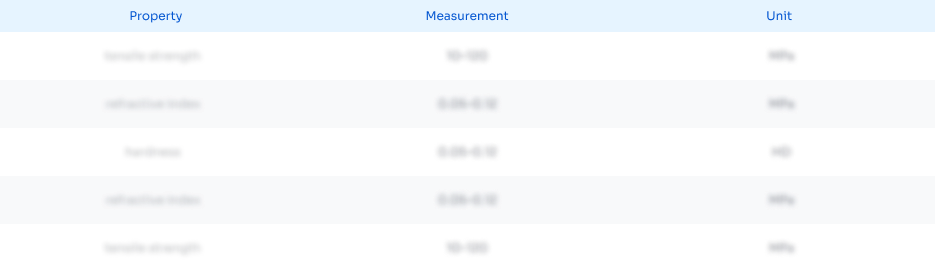
Abstract
Description
Claims
Application Information
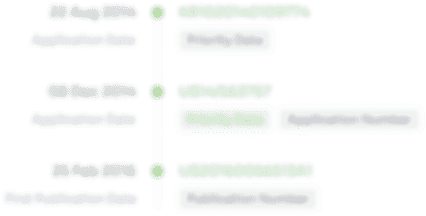
- R&D
- Intellectual Property
- Life Sciences
- Materials
- Tech Scout
- Unparalleled Data Quality
- Higher Quality Content
- 60% Fewer Hallucinations
Browse by: Latest US Patents, China's latest patents, Technical Efficacy Thesaurus, Application Domain, Technology Topic, Popular Technical Reports.
© 2025 PatSnap. All rights reserved.Legal|Privacy policy|Modern Slavery Act Transparency Statement|Sitemap|About US| Contact US: help@patsnap.com