Human body action recognition method fusing attention mechanism and space-time diagram convolutional neural network under security scene
A convolutional neural network and human action recognition technology, applied in the field of human action recognition, can solve problems such as difficulties in data collection and labeling, low frequency of abnormal actions, limited expression ability, etc., to achieve strong generalization ability and enhanced robustness , the effect of strong expressive ability
- Summary
- Abstract
- Description
- Claims
- Application Information
AI Technical Summary
Problems solved by technology
Method used
Image
Examples
Embodiment Construction
[0052] In order to make the features and advantages of this patent more obvious and understandable, the following specific examples are given in conjunction with the drawings, and detailed descriptions are as follows:
[0053] Such as figure 1 As shown, the overall process of this embodiment includes the following steps:
[0054] Step S1: Randomly divide the acquired human motion analysis data set in the security scene into a training set and a verification set;
[0055] In this embodiment, the step S1 specifically includes:
[0056] Step S11: adopt self-built or download public security field data sets; uniformly process the obtained video data, scale the size to 340*256, and adjust the frame rate to 30 frames per second;
[0057] Step S12: The data set is randomly divided into a training set and a validation set according to a ratio of 100:1.
[0058] Step S2: Perform data enhancement processing on the video data of the training set and the verification set;
[0059] In this embodimen...
PUM
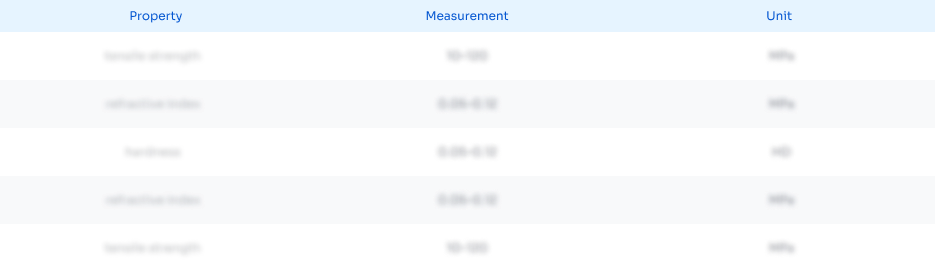
Abstract
Description
Claims
Application Information
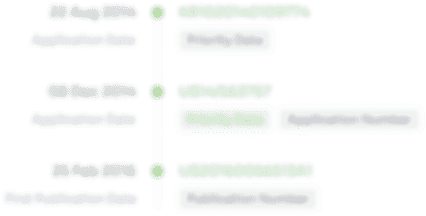
- R&D Engineer
- R&D Manager
- IP Professional
- Industry Leading Data Capabilities
- Powerful AI technology
- Patent DNA Extraction
Browse by: Latest US Patents, China's latest patents, Technical Efficacy Thesaurus, Application Domain, Technology Topic.
© 2024 PatSnap. All rights reserved.Legal|Privacy policy|Modern Slavery Act Transparency Statement|Sitemap