Method for transplanting deep learning network to FPAG platform
A deep learning network and platform technology, applied in the direction of neural learning methods, biological neural network models, architecture with a single central processing unit, etc., can solve the problems of inconvenient application, time-consuming, large amount of computing and storage resources, etc., to achieve relief Insufficient DSP resources and the effect of increased data processing speed
- Summary
- Abstract
- Description
- Claims
- Application Information
AI Technical Summary
Problems solved by technology
Method used
Image
Examples
Embodiment
[0029] The FPGA platform in this example refers to an integrated look-up table (LTU), flip-flop (FF), digital processing unit (DSP), storage unit RAM and phase-locked loop PLL, and uses AXI bus for on-chip and off-chip data transmission system. The embodiment of the present invention is described by taking such a system as an example to optimize the binary quantization and transplantation acceleration of the VGG model, but the present invention is not limited thereto.
[0030] attached figure 1 It is the FPGA transplantation and optimization method flowchart of the binary deep learning network of the embodiment of the present invention, and the present invention is according to the appended figure 1 The processing flow transplants and optimizes the VGG model. Proceed as follows:
[0031] A. Perform binary quantization on the original VGG model. In this embodiment, the 32-bit floating-point parameters of the original VGG model are quantized and trained as 1-bit fixed-point...
PUM
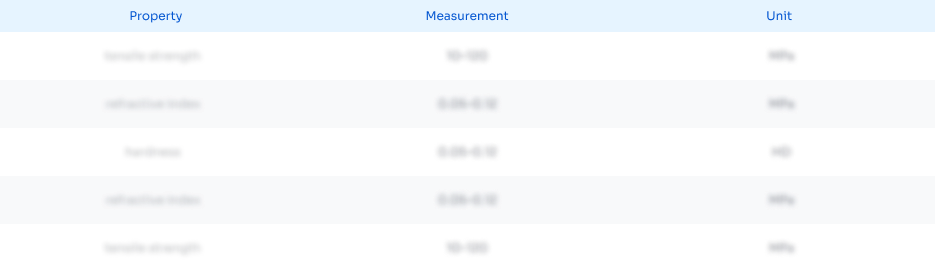
Abstract
Description
Claims
Application Information
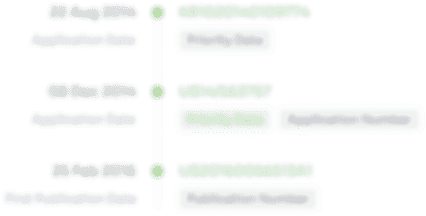
- R&D Engineer
- R&D Manager
- IP Professional
- Industry Leading Data Capabilities
- Powerful AI technology
- Patent DNA Extraction
Browse by: Latest US Patents, China's latest patents, Technical Efficacy Thesaurus, Application Domain, Technology Topic.
© 2024 PatSnap. All rights reserved.Legal|Privacy policy|Modern Slavery Act Transparency Statement|Sitemap