A power electronic circuit fault diagnosis method based on an optimized deep belief network
A technology of power electronic circuits and deep belief networks, which is applied in the field of fault diagnosis of power electronic circuits based on optimized deep belief networks, can solve problems such as inability to effectively distinguish, difficulty in parameter adjustment of fault feature vectors, loss of effective fault information, etc., to achieve improved Classification accuracy and fault tolerance, improving the amount of fault feature data and fault mode recognition accuracy, and avoiding the effect of over-learning
- Summary
- Abstract
- Description
- Claims
- Application Information
AI Technical Summary
Problems solved by technology
Method used
Image
Examples
Embodiment Construction
[0052] Below in conjunction with specific embodiment and accompanying drawing, the present invention will be further described:
[0053] In the embodiment of the present invention, the single-phase five-level inverter is taken as an example, and the single-phase five-level inverter fault diagnosis method based on the optimized deep belief network, referring to the attached figure 1 , including the following steps:
[0054] (1) In order to verify the designed fault diagnosis method, non-real-time offline simulation is a commonly used method, but the main disadvantage of this method is that there will be large There are many uncertain factors. In order to make the fault diagnosis algorithm more practical, the half-physical simulator RT-LAB is used to perform fault injection experiments on the actual prototype. The RT-LAB half-physical simulation platform is a set of industrial-grade system real-time simulation platform launched by Canada's Opal-RT Technologies. , can directly ...
PUM
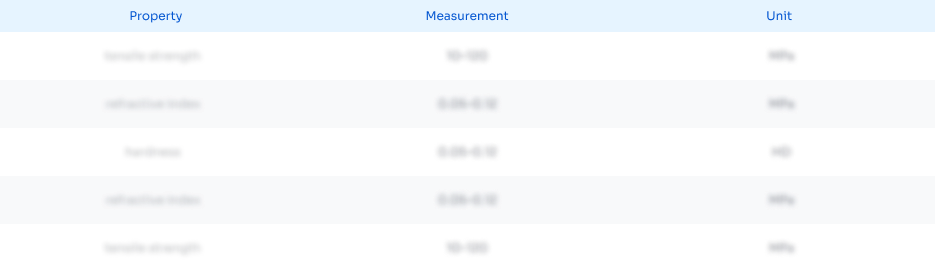
Abstract
Description
Claims
Application Information
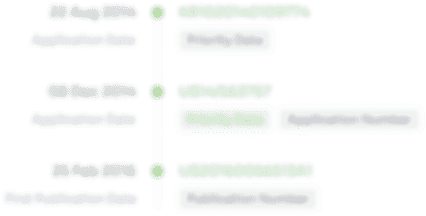
- R&D Engineer
- R&D Manager
- IP Professional
- Industry Leading Data Capabilities
- Powerful AI technology
- Patent DNA Extraction
Browse by: Latest US Patents, China's latest patents, Technical Efficacy Thesaurus, Application Domain, Technology Topic, Popular Technical Reports.
© 2024 PatSnap. All rights reserved.Legal|Privacy policy|Modern Slavery Act Transparency Statement|Sitemap|About US| Contact US: help@patsnap.com