Human body behavior identification method based on Bi-LSTM-Attention model
A recognition method and model technology, used in character and pattern recognition, neural learning methods, biological neural network models, etc., can solve problems such as easy underfitting, inability to effectively learn motion time series features, and difficulty in optimizing network models due to gradient dissipation. , to achieve good performance
- Summary
- Abstract
- Description
- Claims
- Application Information
AI Technical Summary
Problems solved by technology
Method used
Image
Examples
Embodiment Construction
[0037] The present invention will be further described below in conjunction with specific drawings and embodiments.
[0038] The present invention proposes a human action recognition method based on Bi-LSTM-Attention model (One HumanAction Recognition Algorithm Based on Bi-LSTM-Attention model);
[0039]The method first extracts 20 video frames from each video, extracts the depth features of the video frames through the InceptionV3 model, and then constructs the feature vectors in the forward and backward Bi-LSTM neural networks, and then uses the attention (Attention) mechanism The model adaptively perceives the network weights that have a greater impact on the recognition results, so that the Bi-LSTM-Attention model can achieve more accurate recognition according to the context of the behavior, and finally classify the video through a fully connected layer connected to the softmax classifier .
[0040] The method mainly includes three steps:
[0041] Step S1, input the ext...
PUM
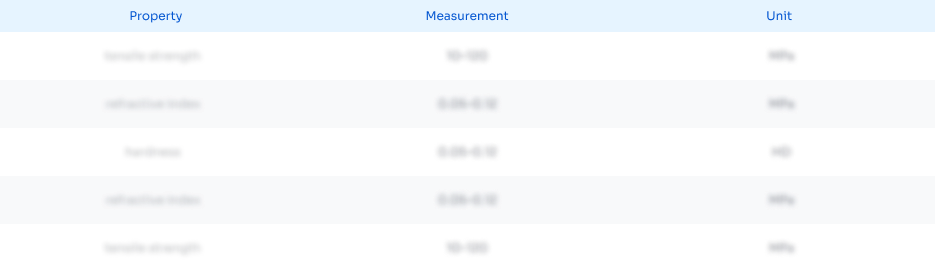
Abstract
Description
Claims
Application Information
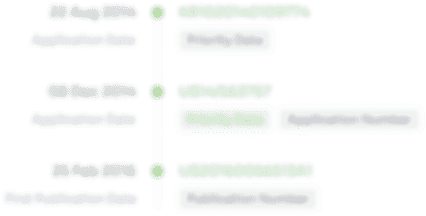
- Generate Ideas
- Intellectual Property
- Life Sciences
- Materials
- Tech Scout
- Unparalleled Data Quality
- Higher Quality Content
- 60% Fewer Hallucinations
Browse by: Latest US Patents, China's latest patents, Technical Efficacy Thesaurus, Application Domain, Technology Topic, Popular Technical Reports.
© 2025 PatSnap. All rights reserved.Legal|Privacy policy|Modern Slavery Act Transparency Statement|Sitemap|About US| Contact US: help@patsnap.com