Joint learning method for features and strategies based on state features and subsequent features
A technology of state characteristics and learning methods, applied in the field of deep reinforcement learning, can solve problems such as low sample utilization, affect network training and convergence, increase network training costs, etc., to improve sample utilization efficiency, efficient policy learning, and learning speed accelerated effect
- Summary
- Abstract
- Description
- Claims
- Application Information
AI Technical Summary
Problems solved by technology
Method used
Image
Examples
Embodiment Construction
[0015] The technical solutions in the embodiments of the present invention will be clearly and completely described below in conjunction with the accompanying drawings in the embodiments of the present invention. Obviously, the described embodiments are only some of the embodiments of the present invention, not all of them. Based on the embodiments of the present invention, all other embodiments obtained by persons of ordinary skill in the art without making creative efforts belong to the protection scope of the present invention.
[0016] The embodiment of the present invention provides a joint learning method of features and strategies based on state features and subsequent features, aiming to solve the problem of low utilization efficiency of feature learning samples in traditional deep reinforcement learning.
[0017] This program analyzes the reinforcement learning formula and designs a policy learning program in combination with a deep network. For the reinforcement lear...
PUM
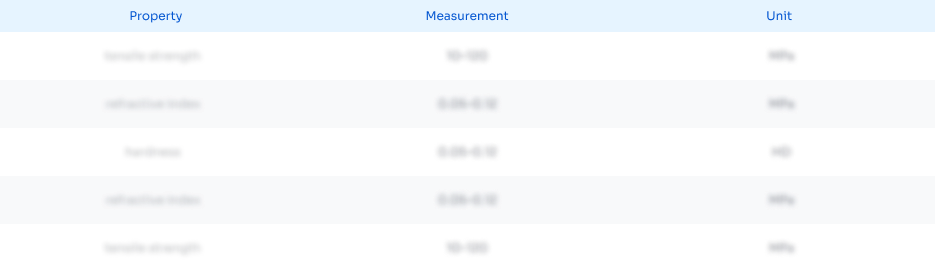
Abstract
Description
Claims
Application Information
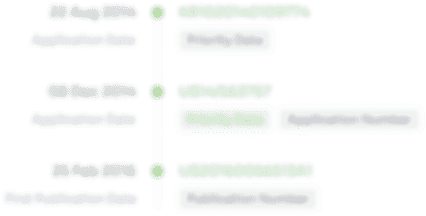
- R&D Engineer
- R&D Manager
- IP Professional
- Industry Leading Data Capabilities
- Powerful AI technology
- Patent DNA Extraction
Browse by: Latest US Patents, China's latest patents, Technical Efficacy Thesaurus, Application Domain, Technology Topic, Popular Technical Reports.
© 2024 PatSnap. All rights reserved.Legal|Privacy policy|Modern Slavery Act Transparency Statement|Sitemap|About US| Contact US: help@patsnap.com