Full convolutional network semantic segmentation method based on multi-scale low-level feature fusion
A fully convolutional network and multi-scale feature technology, applied in the field of full convolutional network semantic segmentation, can solve problems such as rough segmentation of objects and inability to recognize small-scale objects, and solve the problem of edge blurring, improve recognition effects, and strengthen The effect of sensitivity
- Summary
- Abstract
- Description
- Claims
- Application Information
AI Technical Summary
Problems solved by technology
Method used
Image
Examples
Embodiment
[0032] Such as figure 1 as shown, figure 1 It is a flowchart of an embodiment of the fully convolutional neural network based on multi-scale low-level feature fusion of the present invention. This embodiment comprises the following steps:
[0033] 1) Use a fully convolutional neural network to extract dense features from the input image;
[0034] 2) Perform multi-scale feature fusion processing on the extracted features;
[0035] 3) The image after multi-scale feature fusion is processed through 3×3 convolution layer, category convolution layer and bilinear interpolation upsampling to obtain a score map of the same size as the original image, so as to realize the semantic segmentation task of the image.
[0036] Image semantic segmentation is a typical problem of predicting the semantic category of each pixel through dense feature extraction. Therefore, to improve the category prediction accuracy of each pixel, it is necessary to use global and fine feature expression. The...
PUM
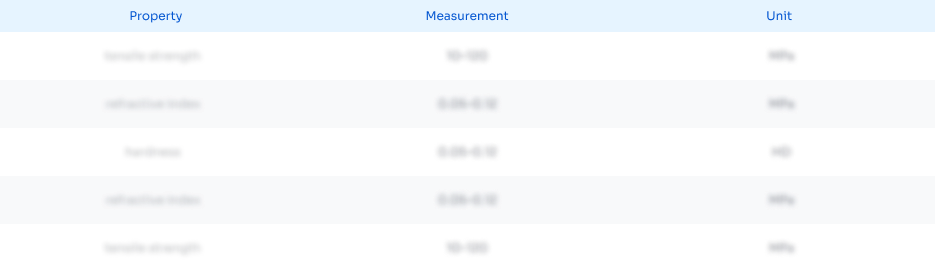
Abstract
Description
Claims
Application Information
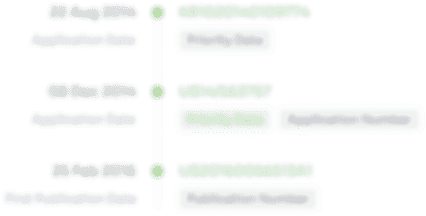
- R&D Engineer
- R&D Manager
- IP Professional
- Industry Leading Data Capabilities
- Powerful AI technology
- Patent DNA Extraction
Browse by: Latest US Patents, China's latest patents, Technical Efficacy Thesaurus, Application Domain, Technology Topic, Popular Technical Reports.
© 2024 PatSnap. All rights reserved.Legal|Privacy policy|Modern Slavery Act Transparency Statement|Sitemap|About US| Contact US: help@patsnap.com