A dilated fully convolutional neural network device and its construction method
A technology of convolutional neural network and construction method, which is applied in the field of image signal processing, can solve problems such as unsmooth results, discontinuous pixels, rough result maps, etc., and achieve the goal of solving labeling problems, less model parameters, and simple model structure Effect
- Summary
- Abstract
- Description
- Claims
- Application Information
AI Technical Summary
Problems solved by technology
Method used
Image
Examples
Embodiment 1
[0044] figure 1 It is a simplified diagram of the expanded full convolutional network structure disclosed in the present invention. The network consists of three parts, including the convolutional neural network part, feature extraction module, and feature fusion module. The convolutional layer in the figure is represented as "Conv", and "Pooling" represents the pooling layer.
[0045] (1) Convolutional neural network:
[0046] Convolutional neural network can select all existing convolutional neural networks, including VGG-Net, ResNet, DenseNet, etc. Convolutional neural network is a network used for image classification, generally consisting of some convolutional layers, pooling layers and full Connection layer composition, when we build a full convolutional network, we need to remove the last fully connected layer and classification layer in the convolutional network for classification, leaving only the middle convolutional layer and pooling layer, and from these middle l...
PUM
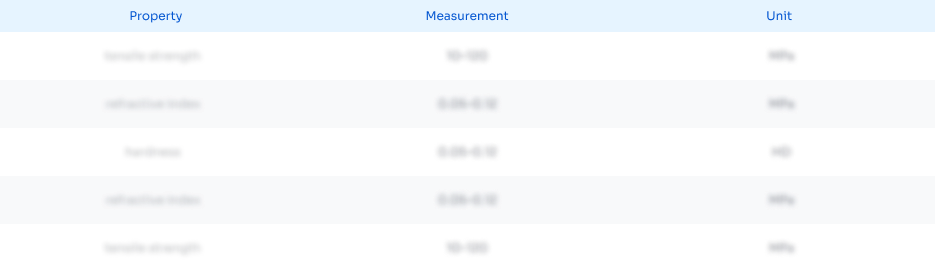
Abstract
Description
Claims
Application Information
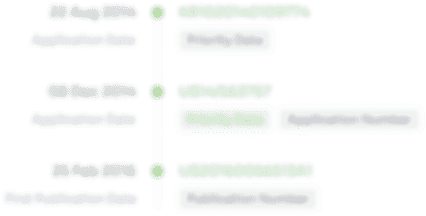
- R&D Engineer
- R&D Manager
- IP Professional
- Industry Leading Data Capabilities
- Powerful AI technology
- Patent DNA Extraction
Browse by: Latest US Patents, China's latest patents, Technical Efficacy Thesaurus, Application Domain, Technology Topic, Popular Technical Reports.
© 2024 PatSnap. All rights reserved.Legal|Privacy policy|Modern Slavery Act Transparency Statement|Sitemap|About US| Contact US: help@patsnap.com