A machine learning-based mimo link adaptive transmission method
A link adaptive and machine learning technology, applied in the field of wireless communication, can solve problems such as system complexity and dimension increase, inability to effectively describe MIMO or OFDM systems, and SNR inability to provide sufficient transmission modes, etc. Achieve the effect of reducing feature dimension and computational complexity
- Summary
- Abstract
- Description
- Claims
- Application Information
AI Technical Summary
Problems solved by technology
Method used
Image
Examples
Embodiment Construction
[0027] The technical solution of the present invention will be further introduced below in combination with specific embodiments.
[0028] This specific embodiment discloses a MIMO link adaptive transmission method based on machine learning, including the following steps:
[0029] S1: Use machine learning unsupervised learning algorithm to extract features of channel state information. Channel state information includes channel quality indication and rank indication. Unsupervised learning algorithms are self-encoding structures that fit data by using a multi-layer neural network. Among them, the self-encoding structure is realized through the following process: using the training data without class labels, under the condition of adding sparsity and the limitation of the number of neural units, try to approximate an identity function, and perform feature extraction and dimensionality reduction on the original input data. For example, the identity function is h W,b (x)≈x, whe...
PUM
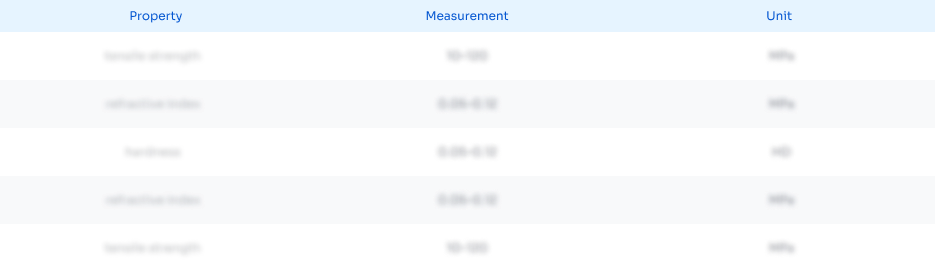
Abstract
Description
Claims
Application Information
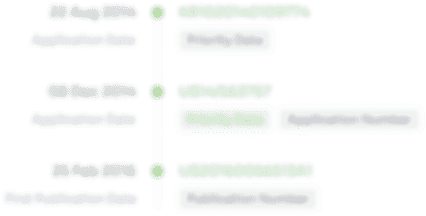
- Generate Ideas
- Intellectual Property
- Life Sciences
- Materials
- Tech Scout
- Unparalleled Data Quality
- Higher Quality Content
- 60% Fewer Hallucinations
Browse by: Latest US Patents, China's latest patents, Technical Efficacy Thesaurus, Application Domain, Technology Topic, Popular Technical Reports.
© 2025 PatSnap. All rights reserved.Legal|Privacy policy|Modern Slavery Act Transparency Statement|Sitemap|About US| Contact US: help@patsnap.com