Multi-model cooperative defense method facing deep learning antagonism attack
A technology of deep learning and collaborative defense, applied in neural learning methods, biological neural network models, character and pattern recognition, etc., can solve problems such as indefensibility and low security
- Summary
- Abstract
- Description
- Claims
- Application Information
AI Technical Summary
Problems solved by technology
Method used
Image
Examples
Embodiment Construction
[0048] The present invention will be further described below in conjunction with the accompanying drawings.
[0049] refer to figure 1 , a multi-model collaborative defense method for deep learning adversarial attacks, including the following steps:
[0050] 1) The ρ-loss model is proposed for unified modeling of gradient-based attacks, and the principle of gradient-based confrontation attacks is further analyzed. The process is as follows:
[0051] 1.1) Unify all gradient-based adversarial sample generation methods into an optimized ρ-loss model, which is defined as follows:
[0052] arg min λ 1 ||ρ|| p +λ 2 Loss(x adv ,f pre (x adv ))s.t.ρ=x nor -x adv (1)
[0053] In formula (1), ρ represents the adversarial sample x adv with normal sample x nor The disturbance existing between; f pre ( ) indicates the predicted output of the deep learning model; ||·|| p Represents the p-norm of the disturbance; Loss(·,·) represents the loss function; λ 1 and lambda 2 Is th...
PUM
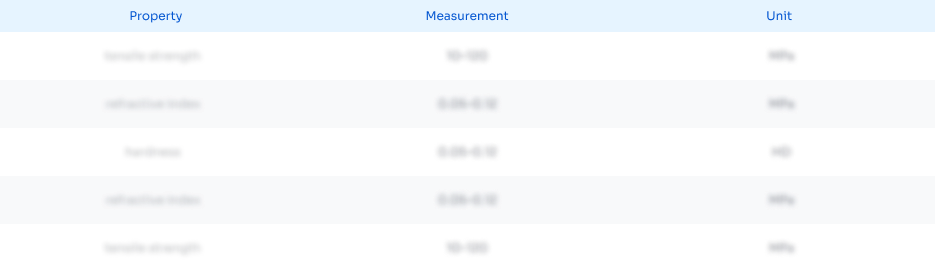
Abstract
Description
Claims
Application Information
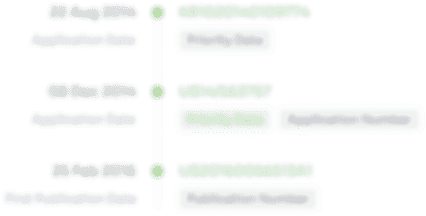
- R&D Engineer
- R&D Manager
- IP Professional
- Industry Leading Data Capabilities
- Powerful AI technology
- Patent DNA Extraction
Browse by: Latest US Patents, China's latest patents, Technical Efficacy Thesaurus, Application Domain, Technology Topic, Popular Technical Reports.
© 2024 PatSnap. All rights reserved.Legal|Privacy policy|Modern Slavery Act Transparency Statement|Sitemap|About US| Contact US: help@patsnap.com