Method and device for quality enhancement of compressed binocular images based on convolutional neural network
A convolutional neural network and image quality technology, applied in the field of image processing, can solve the problems of image quality damage, pixel value discontinuity, and blurring in the middle viewpoint, achieve low transmission and storage costs, avoid excessive smoothing of regions, and expand applications range effect
- Summary
- Abstract
- Description
- Claims
- Application Information
AI Technical Summary
Problems solved by technology
Method used
Image
Examples
Embodiment Construction
[0034] In order to make the object, technical solution and advantages of the present invention clearer, the present invention will be further described in detail below in conjunction with the accompanying drawings and embodiments. It should be understood that the specific embodiments described here are only used to explain the present invention, not to limit the present invention.
[0035] In the prior art, there is a problem that image quality is damaged after binocular images are compressed using an asymmetric lossy compression mode.
[0036] In order to solve the above technical problems, the present invention proposes a method and device for enhancing the quality of compressed binocular images based on a fully convolutional neural network. The fully convolutional neural network provided by the present invention is used to extract high-frequency information in virtual viewpoint images and fuse into the original low-quality image, thereby restoring the information lost in the ...
PUM
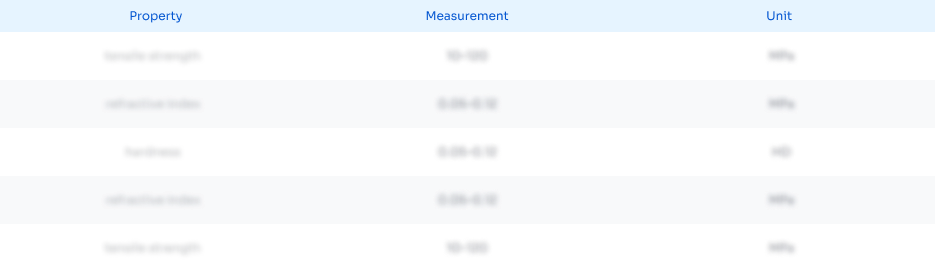
Abstract
Description
Claims
Application Information
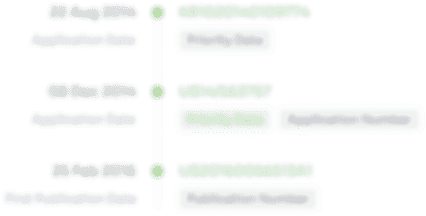
- R&D Engineer
- R&D Manager
- IP Professional
- Industry Leading Data Capabilities
- Powerful AI technology
- Patent DNA Extraction
Browse by: Latest US Patents, China's latest patents, Technical Efficacy Thesaurus, Application Domain, Technology Topic, Popular Technical Reports.
© 2024 PatSnap. All rights reserved.Legal|Privacy policy|Modern Slavery Act Transparency Statement|Sitemap|About US| Contact US: help@patsnap.com