Deep learning network construction method and system applicable to semantic segmentation
A deep learning network, semantic segmentation technology, applied in the field of computer vision, can solve the problem of low accuracy of semantic segmentation, achieve the effect of strong details and shape information, ensure integrity, and strong robustness
- Summary
- Abstract
- Description
- Claims
- Application Information
AI Technical Summary
Problems solved by technology
Method used
Image
Examples
specific Embodiment approach
[0053] Such as figure 2 Shown is a schematic flow chart of a deep learning network construction method suitable for semantic segmentation disclosed in the embodiment of the present invention. The method mainly includes the following steps: 1) data collection and preprocessing; 2) network framework (Convolutional Architecture for Fast Feature Embedding, Caffe) relevant file modification; 3) mean field iterative process; 4) joint training of conditional random field and deconvolution network. Its specific implementation is as follows:
[0054] S1. Perform multi-scale transformation on the images in the data set, wherein the images in the above data set have been marked according to categories;
[0055] Among them, the multi-scale includes 3 scales, which are 0.5, 1, and 1.5 respectively, indicating that the original image is scaled by a corresponding multiple.
[0056] S2. Use the multi-scale transformed image and corresponding marks as the input of the deep learning network,...
PUM
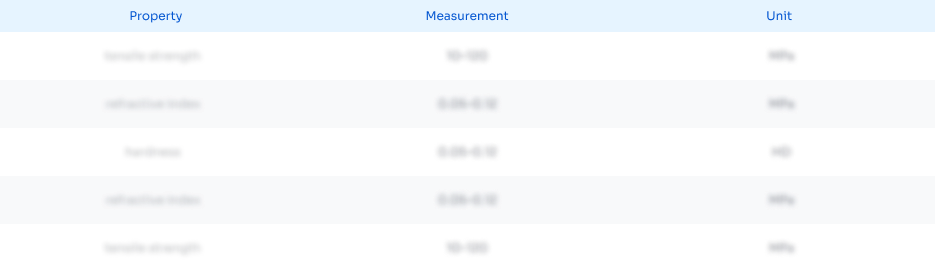
Abstract
Description
Claims
Application Information
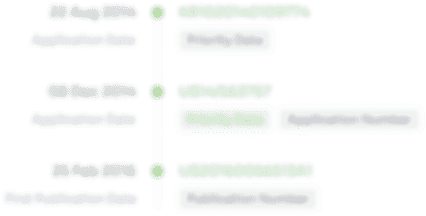
- R&D Engineer
- R&D Manager
- IP Professional
- Industry Leading Data Capabilities
- Powerful AI technology
- Patent DNA Extraction
Browse by: Latest US Patents, China's latest patents, Technical Efficacy Thesaurus, Application Domain, Technology Topic, Popular Technical Reports.
© 2024 PatSnap. All rights reserved.Legal|Privacy policy|Modern Slavery Act Transparency Statement|Sitemap|About US| Contact US: help@patsnap.com