Method for Hash image retrieval based on deep learning and local feature fusion
A local feature and image retrieval technology, applied in computer parts, special data processing applications, instruments, etc., can solve the problems of dissimilarity in local details, large gap in overall outline details, inconsistent results, etc., and achieve fast and efficient image retrieval tasks Effect
- Summary
- Abstract
- Description
- Claims
- Application Information
AI Technical Summary
Problems solved by technology
Method used
Image
Examples
Embodiment Construction
[0039] The present invention will be further described below through specific embodiments.
[0040] figure 1 It is a schematic diagram of the deep learning network structure of the present invention. The network model framework of the present invention is a deep convolutional network based on the improvement of the GoogLeNet network structure, and the deep convolutional network structure is as follows figure 1 As shown, the network consists of five parts: input part, convolutional subnetwork part, local feature fusion part, hash layer coding part and loss function part. The input part contains images and corresponding labels, and the images are input in the form of triplets; the convolutional subnetwork part uses the convolutional part of the GoogLeNet network, and contains the original 3 loss layers; the local feature fusion module is mainly composed of convolution Layer and pooling layer, a merge layer and a fully connected layer; the coding part of the hash layer is compo...
PUM
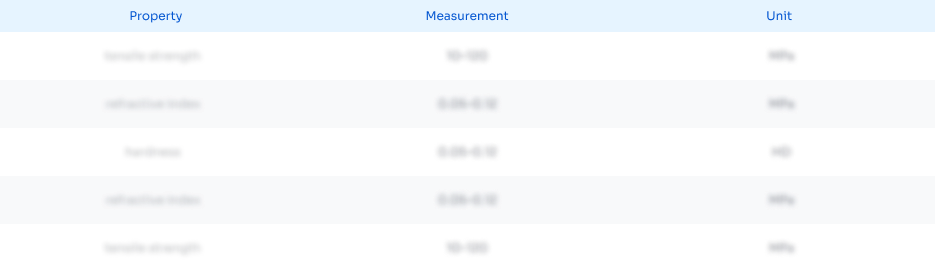
Abstract
Description
Claims
Application Information
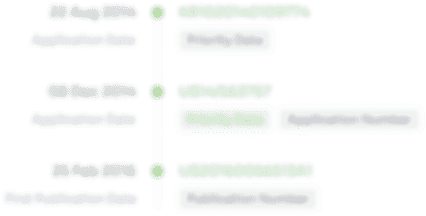
- R&D Engineer
- R&D Manager
- IP Professional
- Industry Leading Data Capabilities
- Powerful AI technology
- Patent DNA Extraction
Browse by: Latest US Patents, China's latest patents, Technical Efficacy Thesaurus, Application Domain, Technology Topic, Popular Technical Reports.
© 2024 PatSnap. All rights reserved.Legal|Privacy policy|Modern Slavery Act Transparency Statement|Sitemap|About US| Contact US: help@patsnap.com