Data-driven representation and clustering discretization method and system for design optimization and/or performance prediction of material systems and applications of same
a data-driven representation and clustering technology, applied in the field of materials, can solve the problems of large computational resources, high cost, and long execution time of finite element software, and achieve the effect of validating the efficiency and accuracy of multi-scale modeling
- Summary
- Abstract
- Description
- Claims
- Application Information
AI Technical Summary
Benefits of technology
Problems solved by technology
Method used
Image
Examples
example 1
Clustering Discretization Methods for Generation of Material Performance Databases in Machine Learning and Design Optimization
[0356]Mechanical science and engineering can use machine learning. However, data sets have remained relatively scarce; fortunately, known governing equations can supplement these data. This exemplary study summarizes and generalizes three reduced order methods: self-consistent clustering analysis, virtual clustering analysis, and FEM-clustering analysis. These approaches have two-stage structures: unsupervised learning facilitates model complexity reduction and mechanistic equations provide predictions. These predictions define databases appropriate for training neural networks. The feed forward neural network solves forward problems, e.g., replacing constitutive laws or homogenization routines. The convolutional neural network solves inverse problems or is a classifier, e.g., extracting boundary conditions or determining if damage occurs. In this example, we...
example 2
Data Science for Finite Strain Mechanical Science of Ductile Materials
[0476]In this example, a mechanical science of materials, based on data science, is formulated to predict process-structure-property-performance relationships. Sampling techniques are used to build a training database, which is then compressed using unsupervised learning methods, and finally used to generate predictions by means of mechanistic equations. The method presented in this example relies on an a priori deterministic sampling of the solution space, a K-means clustering method, and a mechanistic Lippmann-Schwinger equation solved using a self-consistent scheme. This method is formulated in a finite strain setting in order to model the large plastic strains that develop during metal forming processes. An efficient implementation of an inclusion fragmentation model is introduced in order to model this micromechanism in a clustered discretization. With the addition of a fatigue strength prediction method also...
example 3
Predictive Multiscale Modeling for Unidirectional Carbon Fiber Reinforced Polymers
[0537]This exemplary study presents a predictive multiscale modeling scheme for Unidirectional (UD) Carbon Fiber Reinforced Polymers (CFRP). A bottom-up modeling procedure is discussed for predicting the performance of UD structures. UD material responses are computed from high fidelity Representative Volume Elements (RVEs). A data-driven Reduced Order Modeling (ROM) approach compresses RVEs into Reduced Order Models so material responses can be computed in a concurrent fashion along with the structural level simulation. The approach presented in this example is validated against experimental data and is believed to provide design guidance for future fiber reinforced polymers development process.
[0538]In modern engineering applications, composite materials are receiving growing attention for their extraordinary lightweight and strength. To understand the mechanical performance of various CFRP designs, ...
PUM
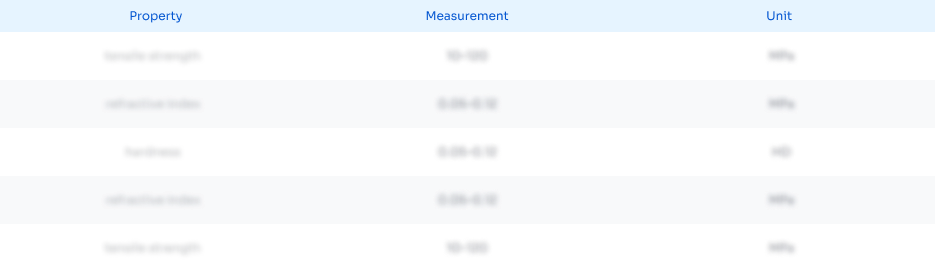
Abstract
Description
Claims
Application Information
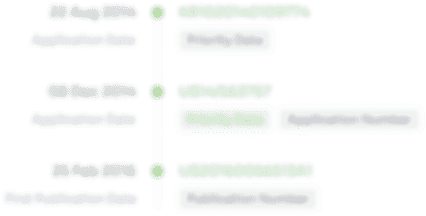
- R&D Engineer
- R&D Manager
- IP Professional
- Industry Leading Data Capabilities
- Powerful AI technology
- Patent DNA Extraction
Browse by: Latest US Patents, China's latest patents, Technical Efficacy Thesaurus, Application Domain, Technology Topic, Popular Technical Reports.
© 2024 PatSnap. All rights reserved.Legal|Privacy policy|Modern Slavery Act Transparency Statement|Sitemap|About US| Contact US: help@patsnap.com