Piecewise linear neuron modeling
a neuron modeling and piecewise technology, applied in the field of artificial nervous systems, can solve problems such as inability to find exact solutions, and achieve the effect of accurate modeling of dynamics and low complexity
- Summary
- Abstract
- Description
- Claims
- Application Information
AI Technical Summary
Benefits of technology
Problems solved by technology
Method used
Image
Examples
example 1
Taylor Expansion for the Izhikevich (Simple) Model
[0164]In this example, Eq. (68) is evaluated for the simple model and linearization based on the Taylor expansion method. To simplify the formulas somewhat, it is assumed that the external current is not present.
[0165]First, the coefficients of the Taylor expansion for the simple model, i.e., F(v)=k(v−vr)(v−vt), are derived starting from Eq. (56). Provided that
1C∂F(v)∂v|vn=2kCvn-kC(vr+vt)(69)
one obtains
a11[vn,nT]=2kCvn-kC(vr+vt)+1C∑i=1Lgi(nT)[hi′(vn)(Ei-vn)-hi(vn)](70)b1[vn,t]=Γ(vn,t)-a11[vn,nT]vn=1C{k(vn-vr)(vn-vt)+∑i=1Lgi(t)hi(vn)(Ei-vn)}-{2kCvn2-kC(vr+vt)vn+1C∑i=1Lgi(nT)[hi′(vn)(Ei-vn)-hi(vn)]vn}(71)
[0166]For non-NMDA synaptic channels, Eqs. (70) and (71) simplify to
a11[vn,nT]=2kCvn-kC(vr+vt)-1C∑i=1Lgi(nT)(72)b1[vn,nT]=1C{-kvn2+kvrvt+∑i=1Lgi(nT)vn}+1C∑i=1Lgi(t)(Ei-vn)(73)
[0167]Taking
b0=1C{-kvn2+kvrvt+∑i=1Lgi(nT)[hi′(vn)(Ei-vn)-hi(vn)]vn}
Eq. (68) now becomes
x(nT+T)=AnTx(nT)+An-1(AnT-I)[b0b2]+∑i=1L[hi(vn)(Ei-vn)]∫0TAnτgi(nT+T-τ)τ[10...
example 2
Subthreshold Dynamics of the Hunzinger Cold Model
[0171]Another example is developed in an effort to examine the subthreshold dynamics of the simple intrinsic conductance model known as the Hunzinger Cold model. In this example, no synaptic currents and the simple, but interesting case of impulsive external current are assumed.
[0172]In the Hunzinger Cold model (see Eq. (22) above) when the membrane voltage is below threshold, the matrix, An is constant and equal to A−:
A-≡[-1 / τ--1 / Cab-a]
[0173]The derivation can be further simplified if the first state variable is defined as the membrane voltage minus the reference voltage. With such a definition, all the constant terms are equal to zero, and Eq. (61) simplifies to
x(t)=A-(t-Tn)x(Tn)+1C∫TntA-(t-τ)Iext(τ)τ[10](77)
[0174]Furthermore, if the external current is assumed to be a Dirac delta function at time Tn with amplitude I, i.e., Iext=(t)+Iδ(t−Tn), then
x(t)=A-(t-Tn)(x(Tn)+1C[10])(78)
[0175]Note that impulsive inputs have the same effect on...
example 3
Approximation with a Backward Rectangular Rule
[0189]To simplify the exposition and notations, this example assumes that the synaptic current does not contain voltage-dependent conductance channels (e.g., NMDA channels) and that there is no external current Iext(t). The derivation is obtained for fixed step sizes of length T. To begin, Eq. (68) may be simplified as follows:
x(nT+T)=AnTx(nT)+q+1C∫0TAnτ∑i=1Lgi(nT+T-τ)(Ei-v(nT+T))τ[10](98)
where the vector q contains constant terms, namely
q=An-1(AnT-I)[b0b2](99)
[0190]For notational convenience, the following vector and matrix may also be defined:
b≡[10]B≡[1000](100)
such that Eq. (98) may be rewritten as
x(nT+T)=AnTx(nT)+1C∫0TAnT∑i=1Lgi(nT+T-τ)Eiτb-1C∫0TAnτ∑i=1Lgi(nT+T-τ)τB·x(nT+T)+q(101)
[0191]Now, the solution is derived for gi(t) modeled as simple exponentials per Eq. (29). In this case, one has
x(nT+T)=AnTx(nT)+1C∑i=1Lgi(nT)Ei∫0TAnτ-(T-τ) / τiτ·b-1C∑i=1Lgi(nT)∫0TAnτ-(T-τ) / τiτB·x(nT+T)+q(102)
[0192]The integrals can be readily solved, and if o...
PUM
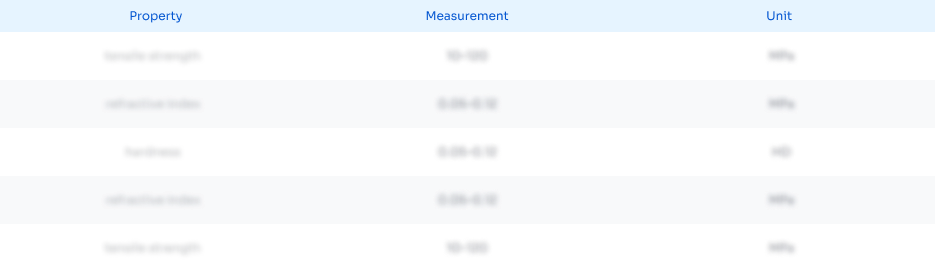
Abstract
Description
Claims
Application Information
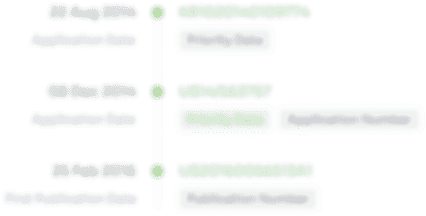
- R&D Engineer
- R&D Manager
- IP Professional
- Industry Leading Data Capabilities
- Powerful AI technology
- Patent DNA Extraction
Browse by: Latest US Patents, China's latest patents, Technical Efficacy Thesaurus, Application Domain, Technology Topic, Popular Technical Reports.
© 2024 PatSnap. All rights reserved.Legal|Privacy policy|Modern Slavery Act Transparency Statement|Sitemap|About US| Contact US: help@patsnap.com