Methods for analysis of spectral data and their applications
a spectral data and analysis method technology, applied in the field of methods and chemometric methods, can solve the problems of large range of desired effects and unwanted side effects in patients, high operational cost of genomic methods, and high complexity of biological consequences of gene expression or gene expression alteration following perturbation
- Summary
- Abstract
- Description
- Claims
- Application Information
AI Technical Summary
Benefits of technology
Problems solved by technology
Method used
Image
Examples
example 1
Identical Versus Non-Identical Twins
[0967] As discussed above, the inventors have developed novel methods (which employ multivariate statistical analysis and pattern recognition (PR) techniques, and optionally data filtering techniques) of analysing data (e.g., NMR spectra) from a test population which yield accurate mathematical models which may subsequently be used to classify a test sample or subject, and / or in diagnosis.
[0968] These techniques have been applied to the analysis of blood serum in the context of identifying identical and non-identical twins. The metabonomic analysis can distinguish between identical and non-identical twins. Novel diagnostic biomarkers for identical and non-identical twins have been identified, and methods for associated classification have been developed.
[0969] This example describes how lifelong differences in metabolism between identical monozygotic (MZ) and non-identical dizygotic (DZ) twins are revealed by 1H NMR based metabonomics, specific...
example 2
Hypertension
[1045] As discussed above, the inventors have developed novel methods (which employ multivariate statistical analysis and pattern recognition (PR) techniques, and optionally data filtering techniques) of analysing data (e.g., NMR spectra) from a test population which yield accurate mathematical models which may subsequently be used to classify a test sample or subject, and / or in diagnosis.
[1046] These techniques have been applied to the analysis of blood serum in the context of hypertension. The metabonomic analysis can distinguish between individuals with and without hypertension. Novel diagnostic biomarkers for hypertension have been identified, and methods for associated diagnosis have been developed.
Obtaining NMR Spectra
[1047] Analysis was performed on serum samples collected as part of the coronary heart disease (CHD) NCA / TVD study described herein.
[1048] The data were classified according to systolic blood pressure (SBP), as follows: [1049] low SBP (≦130 mmHg...
example 3
Diagnosis of Coronary Heart Disease (CHD)
[1105] As discussed above, the inventors have developed novel methods (which employ multivariate statistical analysis and pattern recognition (PR) techniques, and optionally data filtering techniques) of analysing data (e.g., NMR spectra) from a test population which yield accurate mathematical models which may subsequently be used to classify a test sample or subject, and / or in diagnosis.
[1106] In the context of atherosclerosis / CHD, the inventors have applied these techniques to the analysis of either serum or plasma taken from individuals who have been extensively characterized, both for the presence of atherosclerosis / CHD by the gold-standard angiographic technique and also for a wide range of conventional risk factors. The metabonomic analysis can distinguish between individuals with and without atherosclerosis / CHD; and / or the degree of atherosclerosis / CHD. Novel diagnostic biomarkers for atherosclerosis / CHD have been identified, and me...
PUM
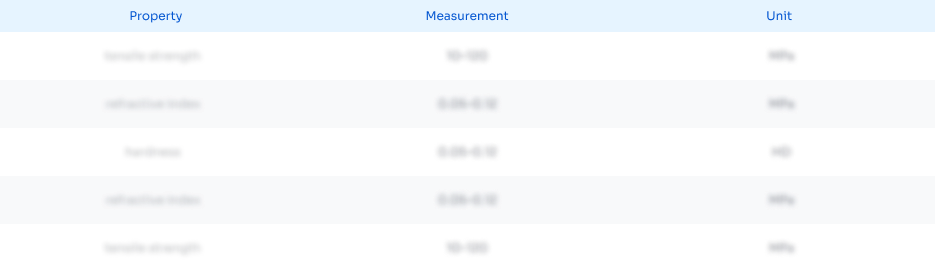
Abstract
Description
Claims
Application Information
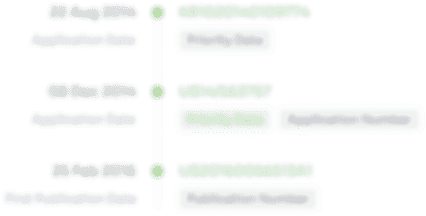
- R&D Engineer
- R&D Manager
- IP Professional
- Industry Leading Data Capabilities
- Powerful AI technology
- Patent DNA Extraction
Browse by: Latest US Patents, China's latest patents, Technical Efficacy Thesaurus, Application Domain, Technology Topic, Popular Technical Reports.
© 2024 PatSnap. All rights reserved.Legal|Privacy policy|Modern Slavery Act Transparency Statement|Sitemap|About US| Contact US: help@patsnap.com