Energy-saving automatic interconnected vehicle service unloading method based on deep reinforcement learning
A technology of reinforcement learning and vehicle service, which is applied in the field of energy-saving automatic interconnected vehicle service offloading, can solve the problems of not considering energy consumption, not conducive to improving the generalization ability of the system, and unloading methods that cannot dynamically balance delay and energy consumption.
- Summary
- Abstract
- Description
- Claims
- Application Information
AI Technical Summary
Problems solved by technology
Method used
Image
Examples
Embodiment Construction
[0096] The present invention will be further described below with reference to the accompanying drawings.
[0097] The present invention proposes an energy-saving automatic interconnected vehicle service offloading method based on deep reinforcement learning, namely a multi-user mode-based asynchronous actor-critic (A3C)-based energy-saving distributed computing offloading method, named ECAC. Like most mainstream reinforcement learning algorithms, ECAC can well adapt to the dynamically changing service scale and type in the Internet of Vehicles environment, and its advantage is that it conforms to the natural distributed system architecture in the Internet of Vehicles environment, and can use each ECD Good model training results can be achieved using only multi-core CPUs (without GPUs). ECAC maps ECDs and agents one-to-one, perfectly mapping A3C to the Internet of Vehicles with device-edge-cloud collaboration. Each ECD collects service requests from connected autonomous vehic...
PUM
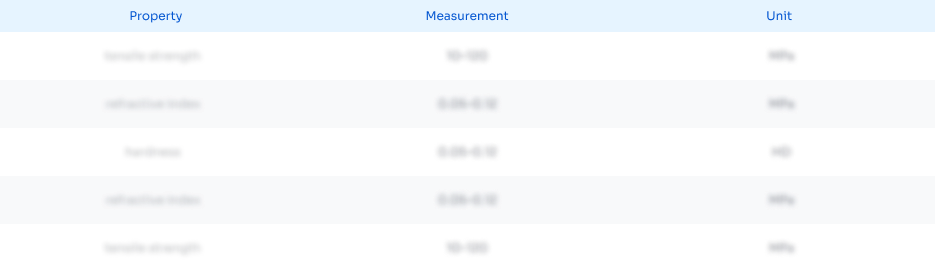
Abstract
Description
Claims
Application Information
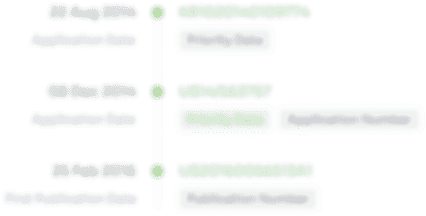
- R&D Engineer
- R&D Manager
- IP Professional
- Industry Leading Data Capabilities
- Powerful AI technology
- Patent DNA Extraction
Browse by: Latest US Patents, China's latest patents, Technical Efficacy Thesaurus, Application Domain, Technology Topic, Popular Technical Reports.
© 2024 PatSnap. All rights reserved.Legal|Privacy policy|Modern Slavery Act Transparency Statement|Sitemap|About US| Contact US: help@patsnap.com