Motor fault diagnosis method based on depth auto-encoder and feature optimization
A self-encoder and feature optimization technology, applied in the field of fault diagnosis, can solve the problems of decreased diagnosis accuracy, insufficient model generalization ability, over-fitting, etc., to improve the running speed and accuracy, improve the fault representation ability, improve The effect of stability
- Summary
- Abstract
- Description
- Claims
- Application Information
AI Technical Summary
Problems solved by technology
Method used
Image
Examples
Embodiment Construction
[0062] Such as figure 1 and 2 As shown, a motor fault diagnosis method based on deep autoencoder and feature optimization includes four processes, as follows:
[0063] Process 1. Signal Processing
[0064] The motor vibration signals collected at different speeds are defined as the source domain and the target domain required by the present invention, wherein the source domain is a marked sample (that is, the state of the motor is known), and the target domain is an unmarked sample. Use DTCWPT to perform signal processing on each sample, decompose it into different grouping nodes, and calculate range, mean, standard deviation, kurtosis, energy, energy entropy, skewness, crest factor, impulse factor, shape factor and latitude Factor 11 statistical parameters to obtain a characteristic data set that characterizes the operating state of the motor. Perform four-layer DTCWPT decomposition on each vibration signal sample in the source domain and the target domain, obtain 16 termi...
PUM
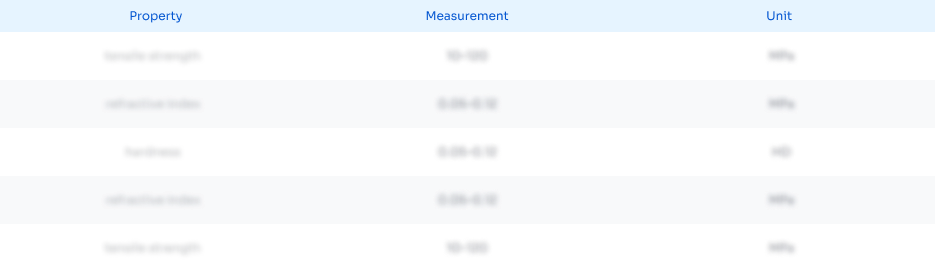
Abstract
Description
Claims
Application Information
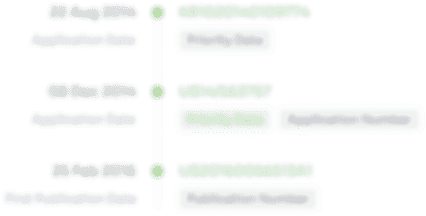
- R&D Engineer
- R&D Manager
- IP Professional
- Industry Leading Data Capabilities
- Powerful AI technology
- Patent DNA Extraction
Browse by: Latest US Patents, China's latest patents, Technical Efficacy Thesaurus, Application Domain, Technology Topic, Popular Technical Reports.
© 2024 PatSnap. All rights reserved.Legal|Privacy policy|Modern Slavery Act Transparency Statement|Sitemap|About US| Contact US: help@patsnap.com