Tire flaw detection method and model based on self-attention mechanism and dual-field self-adaption
A defect detection, dual-domain technology, applied in the field of artificial intelligence transfer learning, can solve the problems of reducing the defect detection performance of deep learning models, large changes in tire defect scale, low contrast, etc., to improve tire defect detection performance, enhance extraction ability, The effect of improving reusability
- Summary
- Abstract
- Description
- Claims
- Application Information
AI Technical Summary
Problems solved by technology
Method used
Image
Examples
Embodiment Construction
[0036] The specific implementation manner of the present invention will be described in detail in conjunction with the accompanying drawings and a specific embodiment of tire defect detection when field deviation exists.
[0037] The specific embodiment selects the two most common defects of foreign matter and bubbles, plus a total of three types of images of normal samples for tire defect detection. The migration scene is migration between different types of X-ray machines, and the source domain samples are sampled from the tire quality inspection line. A model X-ray machine, after cropping, the number of samples of each type is 100, and the size is 224×224. The target domain samples are sampled from another B-type X-ray machine on the quality inspection line. After cropping, 20 samples of each type of unlabeled samples with a size of 224×224 are obtained for training. At the same time, the target domain samples of the same size for testing are 40 for each category, a total o...
PUM
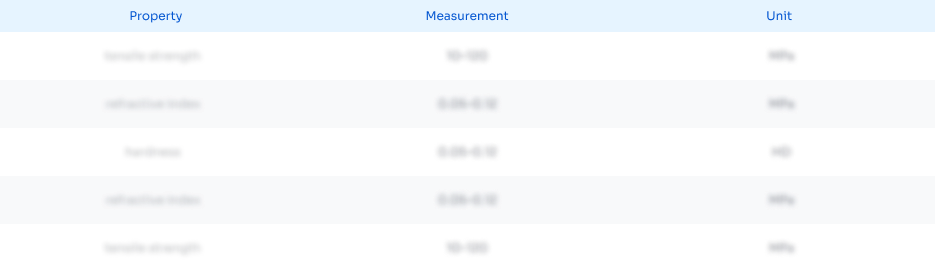
Abstract
Description
Claims
Application Information
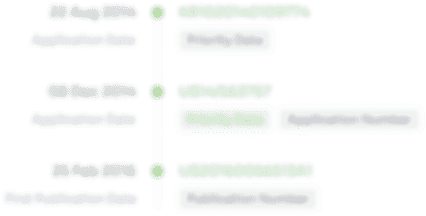
- R&D Engineer
- R&D Manager
- IP Professional
- Industry Leading Data Capabilities
- Powerful AI technology
- Patent DNA Extraction
Browse by: Latest US Patents, China's latest patents, Technical Efficacy Thesaurus, Application Domain, Technology Topic, Popular Technical Reports.
© 2024 PatSnap. All rights reserved.Legal|Privacy policy|Modern Slavery Act Transparency Statement|Sitemap|About US| Contact US: help@patsnap.com