CEEMD-LSTM-MLR-based short-term power load prediction method
A short-term power load and forecasting method technology, applied in forecasting, neural learning methods, based on specific mathematical models, etc., can solve the problems of ignoring the correlation of load data, low forecasting accuracy, etc., to achieve satisfactory forecasting effect, improve forecasting speed, forecasting The effect of improved accuracy
- Summary
- Abstract
- Description
- Claims
- Application Information
AI Technical Summary
Problems solved by technology
Method used
Image
Examples
Embodiment 1
[0034] In this example, the load data at intervals of 15 minutes in the first seven days is used as the model input, and the load data at intervals of 15 minutes in the next day is output as the model. The training set and test set are divided by 7:3, and the load value of the next seven days is predicted. The results of each component of the data decomposed by CEEMD are as follows: figure 2 As shown, the hyperparameters to be optimized in the LSTM model are as follows image 3 As shown, the abscissa represents the forecast date, and the model prediction effect is as follows Figure 4 It can be seen that the predicted results are in good agreement with the real load curve.
PUM
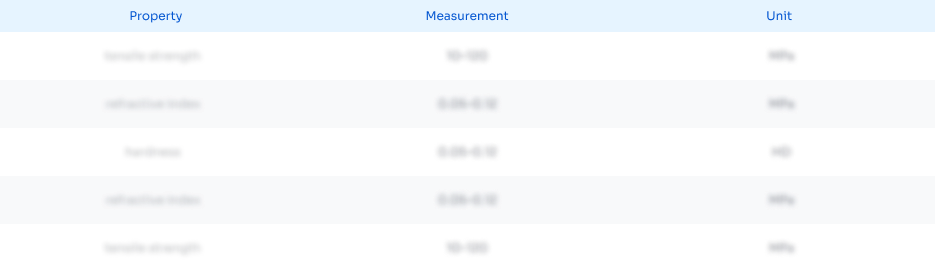
Abstract
Description
Claims
Application Information
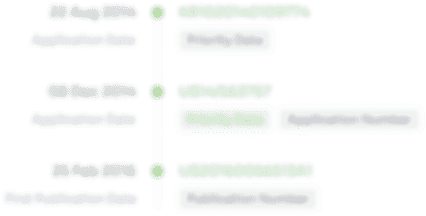
- R&D Engineer
- R&D Manager
- IP Professional
- Industry Leading Data Capabilities
- Powerful AI technology
- Patent DNA Extraction
Browse by: Latest US Patents, China's latest patents, Technical Efficacy Thesaurus, Application Domain, Technology Topic.
© 2024 PatSnap. All rights reserved.Legal|Privacy policy|Modern Slavery Act Transparency Statement|Sitemap