Thyroid cytology multi-type cell detection method based on deep learning
A detection method and deep learning technology, applied in neural learning methods, biological neural network models, image data processing, etc., can solve problems such as missed detection, inapplicable classification of multiple lesion types, single lesion in image blocks, etc., to achieve improved The effect of detection efficiency and reduction of labeling cost
- Summary
- Abstract
- Description
- Claims
- Application Information
AI Technical Summary
Problems solved by technology
Method used
Image
Examples
Embodiment Construction
[0027] Hereinafter, preferred embodiments of the present disclosure will be described in detail with reference to the drawings. In the following description, the same reference numerals are given to the same components, and repeated descriptions are omitted. In addition, the drawings are only schematic diagrams, and the ratio of dimensions between components, the shape of components, and the like may be different from the actual ones.
[0028] It should be noted that the terms "comprising" and "having" and any variations thereof in the present disclosure, such as a process, method, system, product or device that includes or has a series of steps or units, are not necessarily limited to the clearly listed instead, may include or have other steps or elements not explicitly listed or inherent to the process, method, product or apparatus. All methods described in this disclosure can be performed in any suitable order unless otherwise indicated herein or otherwise clearly contradi...
PUM
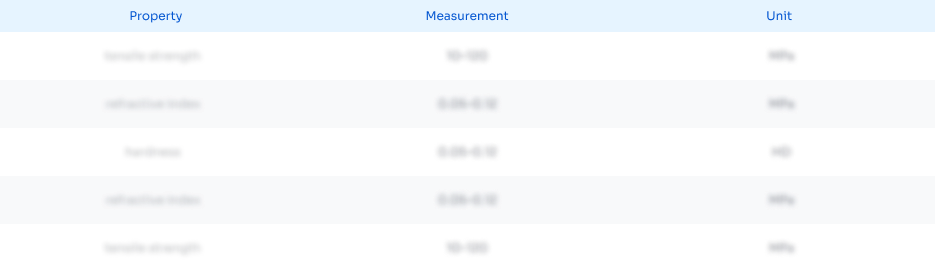
Abstract
Description
Claims
Application Information
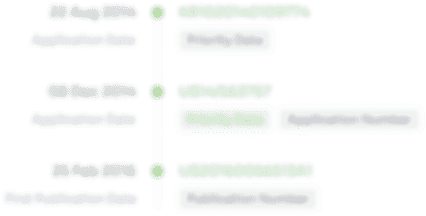
- R&D Engineer
- R&D Manager
- IP Professional
- Industry Leading Data Capabilities
- Powerful AI technology
- Patent DNA Extraction
Browse by: Latest US Patents, China's latest patents, Technical Efficacy Thesaurus, Application Domain, Technology Topic, Popular Technical Reports.
© 2024 PatSnap. All rights reserved.Legal|Privacy policy|Modern Slavery Act Transparency Statement|Sitemap|About US| Contact US: help@patsnap.com